Dieser Inhalt ist nur in englischer Sprache verfügbar.
Recommendation Engine
Click-worthy recommendations
Predict what people need, before they even know they need it. Engage and convert with data and AI.
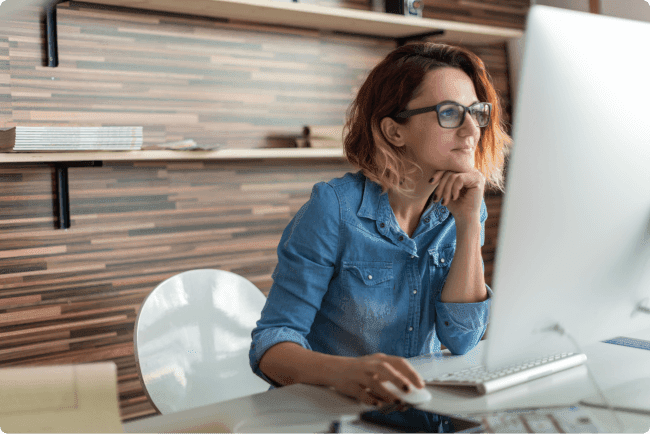
Recommend at every step of the journey
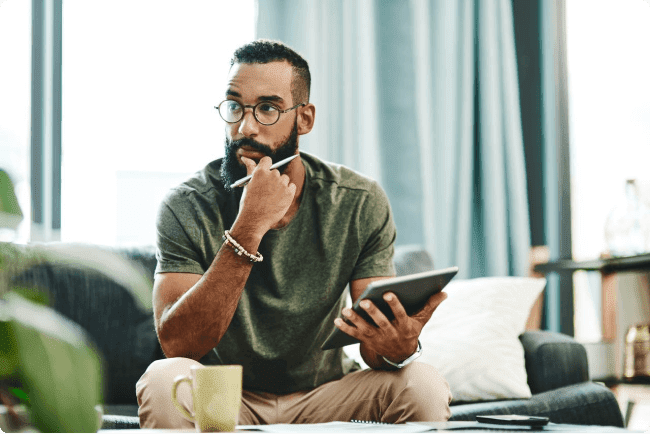
Context-aware recommendations
Show what’s most relevant to a person based on where they are in their journey – from pre-purchase to post-purchase, from seeking answers to exploring content.
Ready-to-go machine learning models
Choose the algorithm that fits your scenario. Coveo provides recommendation models that are easy to set up – including interest-based, frequently viewed and bought together, popular items, and more.
Journey and data stitching
Needs change over time, so do recommendations with Coveo. Connect current context, user history, and more, from across all your data platforms to serve the right content at the right moment.
Powerful, flexible machine learning
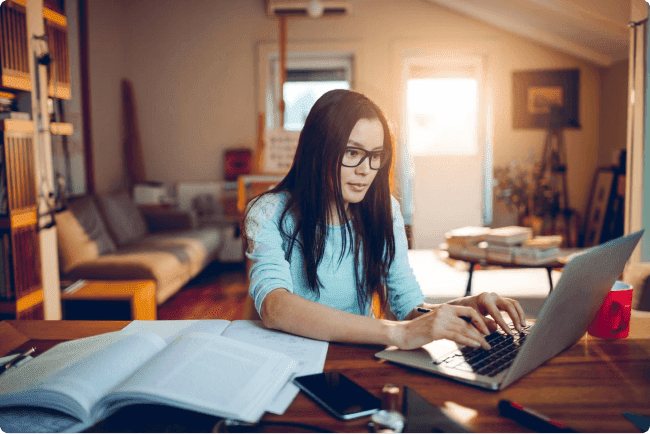
All content types
Define which content types to pull in or create recommendation streams across various content types, and let the recommender carve the most relevant path for each user.
Data set selection
Choose which data sets should train a recommendation model. Focus on a certain region or use inputs from a specific interface – it’s up to you.
Model correction
Draw insights from and respond to your changing product catalog, content, or user behavior. Coveo lets you know when it’s time to retrain your models.
Recommendation experiments
Want to verify which strategy works best? Preview or A/B test different configurations, then optimize and refine.
Start simple and grow your relevance
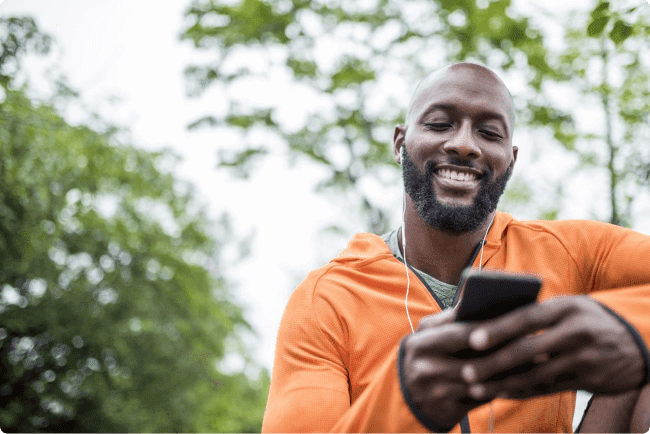
Recommendations from Day 1
Provide search, content, and product recommendations even when data about an item or individual is sparse.
Rich user profiles
Drive ever-more-relevant recommendations as user profiles are dynamically enriched by actions and affinities.
Scale and precision
Massive content libraries and catalogs with millions of SKUs are no problem with Coveo. Our recommendation system zeros in on the right thing for the right person.
Frequently asked questions
We already know that 43% of consumers would pay more if they could find what they’re looking for in just a few clicks (that number increases to 48% for millennials). We also see the 2.5 hours spent per day per employee seeking relevant information. A recommendation engine empowers proactively to find and reveal exactly what your customers and employees want or are likely to need next. See how the Coveo recommendation engine works.
A recommendation engine uses advanced machine learning algorithms to analyze both aggregate and individual user data to deliver the most relevant products and content to the people interacting with it. You’ve likely come across a recommendation engine while shopping online, watching Netflix, or even using a dating app. Learn more about ML-powered recommendation engines.
An AI-powered recommendation engine surfaces the most relevant products and content for each person by learning from what is viewed, clicked, and interacted across similar sessions. Learn more about AI-powered recommendation engines.
The accuracy of product or content recommendations depends on a few important variables. You need data—lots and lots of data. And you need an AI-powered recommendation engine capable of using that data as part of a hybrid filtering method. A hybrid filtering method combines persona-based personalization (collaborative filtering) and learning based on user behavior (content-based filtering), replacing the need for manually hard-coded rules. Again, all of this filtering is based on the engine’s ability to tap into and analyze a wide variety of data sets all at once. Read more best practices for improving the accuracy of recommendations.