Whether engaging customers through chatbots or automating routine processes, organizations today use AI throughout multiple aspects of their business as a critical tool for success. Generative AI implementations like ChatGPT further spurring AI adoption and growth, the need for human expertise and judgment in ensuring the proper functioning of AI is more important than ever before.
“Human in the loop” is an approach that has emerged to integrate human oversight into AI processes. It also acknowledges the necessity of human and machine collaboration in developing and refining a machine learning model for better results. An ML model may display bias or generate misinformation and require further refining.
If your organization has concerns over fairness and accountability, Human in the loop (HITL) may be a solution for you. We’ll take a look at the role of humans in AI automation today — and how businesses can benefit from a human in the loop approach.
What Is Human in the Loop?
Human in the loop (HITL) refers to the integration of human decision making and oversight into an AI model to produce more effective and favorable results. HITL ML is a recognition that while AI displays incredible abilities to perform certain tasks well, human judgment and foresight remain necessary elements in many aspects of decision making and problem solving.
When a machine makes the wrong choice from an ethical standpoint or misinterprets the nuanced meaning behind a phrase, humans need to step in to make the right decisions.
This approach also allows organizations to act on the idea that human expertise and machine intelligence together — produce better results and greater efficiency than what a human or machine can achieve alone. Automated systems may detect potential fraudulent activities in financial services with great speed and precision. However, it is when a human steps in to add expertise in investigating the complexities of a case that can either confirm or reject fraud suspicions.
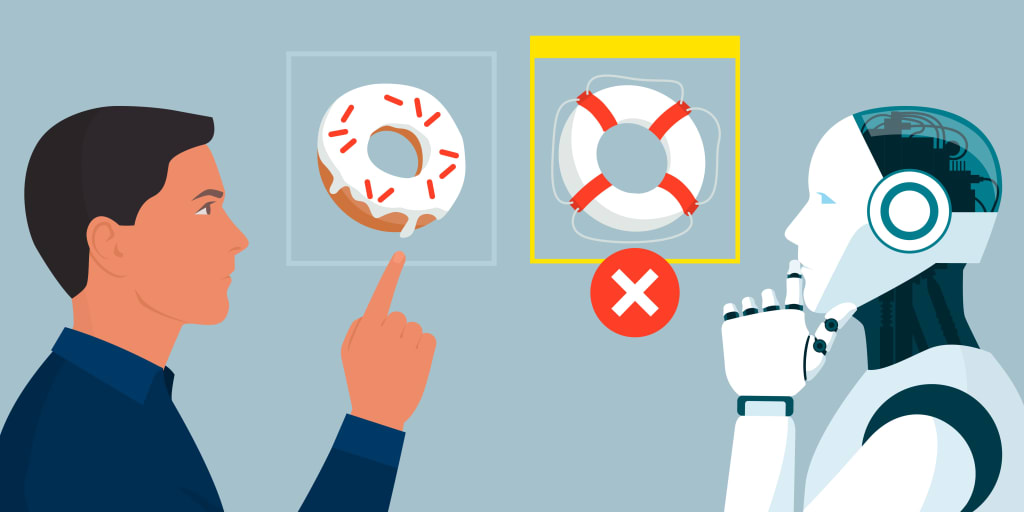
In the context of machine learning, a human in the loop approach brings together the strengths of machine learning and human intellect to build a more effective algorithm that produces better results. Machine learning is a subset of artificial intelligence that teaches computers to learn from algorithms based on vast amounts of data. In HITL machine learning, human intelligence and judgment are necessary to train and develop machine learning models. For example, in the training stage, machines need humans to tell them how to properly identify data and draw the right conclusions from it. Humans help machines become smarter over time in a continuous loop that involves training, testing, tuning and validating.
The need for this approach can be seen starkly in instances of bias in AI algorithms, which in turn can lead to poor and costly decisions for businesses. For example, studies show facial recognition technology, which have been used in law enforcement and hiring decisions, differed in accuracy across demographic groups. A human can potentially identify bias in an AI algorithm and address it before it leads to more serious problems such as damaging a company’s reputation or bottom line.
Role of Humans in Automation
Finding the right balance between human and machine collaboration is key to success as businesses increasingly incorporate AI and automation into their business processes and decision making. The balance becomes even more critical in high-risk situations such as diagnosing patients based on AI-powered evaluations of medical imaging. Let’s take a closer look at the important role of humans in automation systems today.
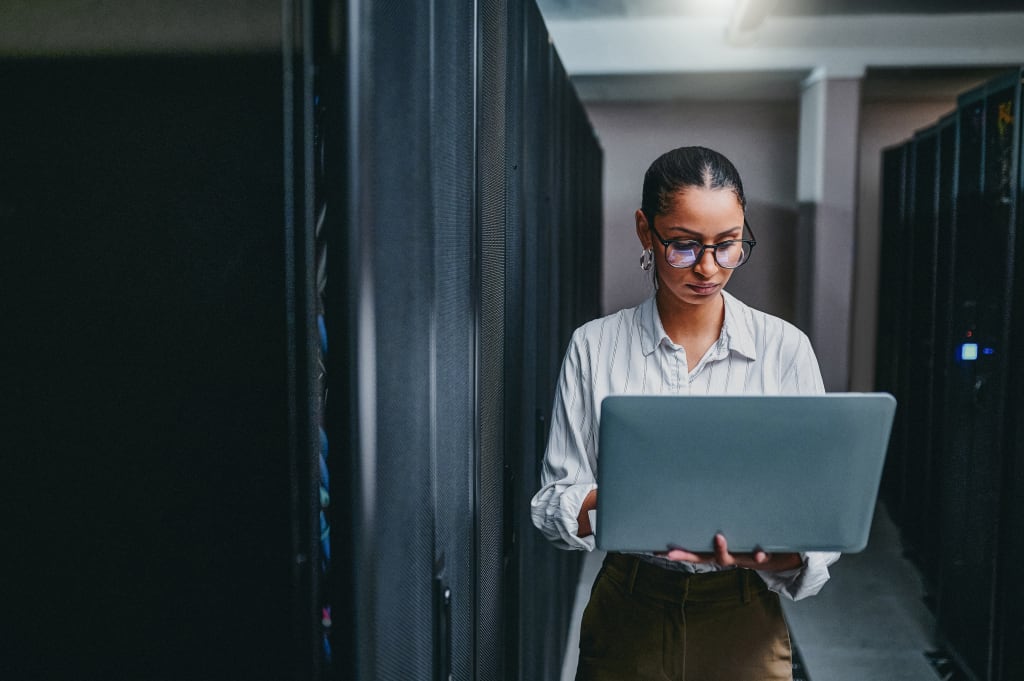
Quality Control and Accuracy
Human reviewers confirm the accuracy and quality of generated outputs from AI or automated systems. For example, in manufacturing, human inspectors may supervise the automated systems responsible for inspecting products and catching defects on the production line to ensure they are meeting the standards of quality.
Decision Making
AI systems could make decisions that are unethical or may encounter ambiguous and complex situations for which they do not have responses. In autonomous driving, for example, the car may face a situation on the road for which it does not have the training to react appropriately and a human needs to intervene. Human judgment is necessary to ensure decisions meet societal and company standards for ethics and values.
Training and Feedback
Humans are crucial to training AI models including labeling and annotating the data, checking performance of the output and tuning algorithms so they improve over time. Global retailer H&M included merchandisers in the testing and development of a pricing algorithm for the end of the season. The combined human and machine workflow, which saw the algorithm working together with the human merchandiser in setting pricing, led to better results than the AI algorithm or humans on their own.
Exception Handling
When an AI system runs into an exceptional situation or one around which there is a scarcity of data, a human must intervene with their knowledge or expertise to guide the situation to an acceptable outcome.
HITL Machine Learning: How Does It Work?
Machine learning involves the development of algorithms that allow computers to learn from data and make predictions without being programmed to do so. The concept of human in the loop recognizes the need to combine AI and human intelligence to build algorithms and create machine learning models. An HITL approach to machine learning maintains that building a foundation of datasets through a team of human data annotators will lead to data that is more accurate, responsible and unbiased.
Implementing human expertise into the development of machine learning models typically involves the following steps:
- Data labeling and training: Humans label data and feed it to machine learning algorithms so they can learn the relationships between data and understand how to apply them to various scenarios.
- Testing and tuning: Humans tune the model in a feedback loop to further develop the algorithm and improve its accuracy.
- Validating: Humans score the model’s outputs and validate them to help the algorithms make the right decisions in situations involving ambiguity or nuances that are difficult for machines to decipher.
Human experts can also play significant roles in the different types of AI learning: supervised and unsupervised learning. Supervised learning involves feeding labeled data into an ML algorithm to make predictions and classify the data. It’s typically an expensive and time-consuming task, but humans can speed it up by injecting human expertise into the labeling process. With unsupervised learning, which involves machines working with unlabeled data to identify patterns and relationships, humans can verify their findings and provide feedback on the outputs.
Significance of HITL for Businesses
While we recognize the impressive abilities of today’s AI models to take large amounts of data and make accurate predictions, businesses must still rely on humans for contextual understanding and judgment in making the right decisions in many scenarios.
Here’s why HITL is so important to businesses:
- Ensuring accuracy and precision: In enterprise settings, small mistakes can incur significant costs. A human in the loop validates AI-generated outputs and maintains precision at the human level in situations that require it such as safety inspections of airplane parts.
- Greater efficiency: AI works better when it complements, rather than supplants, human intelligence. A 2018 Stanford study showed that human workflows that used AI in diagnosing the presence of pneumonia in chest X-rays outperformed diagnoses by human practitioners or AI systems alone.
- Reducing bias: Machine learning models can become biased when they are trained on biased data. Bias is a problem that can lead to long-lasting damage to a brand. A human in the loop can identify bias and address it early before it becomes a bigger problem.
- Supplementing rare data: ML algorithms require vast amounts of data to make accurate predictions, but in some situations the data is just not available. An example is diagnosing a rare medical disease where a limited number of cases are available. Incorporating human knowledge and experience in these situations could help provide training data and lead to more accurate predictions.
- Transparency: Another benefit of a human in the loop design is the AI process becomes more transparent. Involving human judgment throughout the steps of the process can help us understand how decisions are made.
Applications of HITL in Business
Human-machine collaboration will play an important role in further developing and strengthening the use of machine learning in many areas of business. Some key applications for businesses include the following.
Customer Support
We believe generative AI is best suited for handling issues and questions historically taken by Level 1 agents. Human agents are necessary to handle unfamiliar problems, critical issues or more nuanced issues. Machines can augment a human agent’s interactions with customers by equipping the agent with insights tailored to a customer or summarizing information that helps the agent understand an issue more quickly. Human experts are also necessary to train language models on the meaning behind language in their specific domain of customer support.
Knowledge Base
Companies need a strong knowledge practice built out to truly take advantage of language-based AI. Generative AI can help with creating the content necessary to fill out your knowledge base while humans are needed to check for accuracy and relevance before publishing.
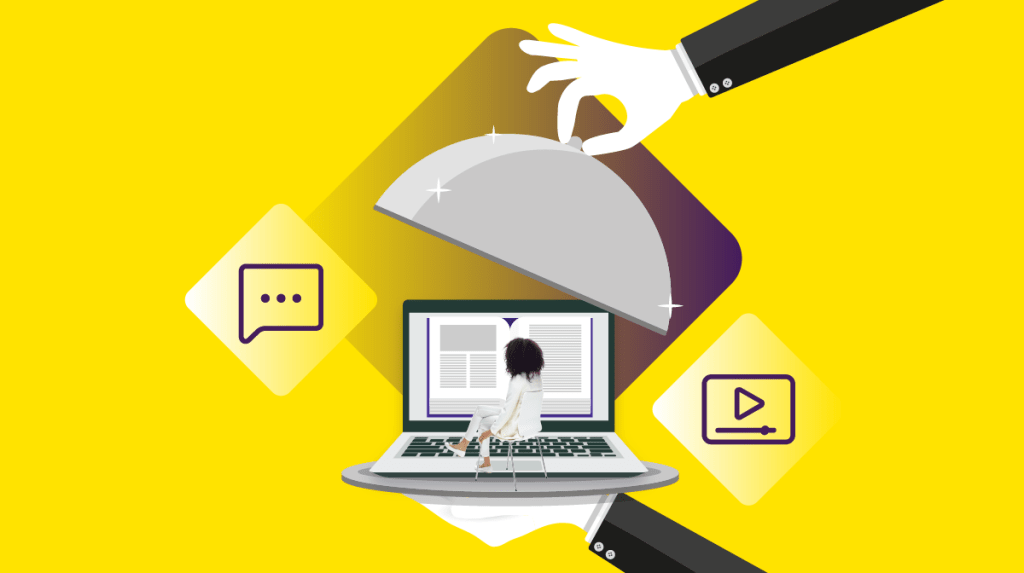
Content Moderation
When it comes to monitoring online user-generated content, both AI and human involvement will lead to the most effective moderation efforts, according to a recent survey by TELUS International. While AI is a great first line of defense in detecting harmful content that goes against a company’s standards or guidelines, humans are needed to handle more contextual decisions based on nuances that AI has a hard time understanding such as the meaning behind particular phrases or images.
Final Thoughts
One of the biggest challenges for businesses in improving their AI outcomes will be in striking the right balance in human-machine collaborations. By combining human expertise and AI-driven automation effectively in their organizations, companies can address complex, nuanced scenarios while making better decisions that lead to new opportunities for innovation and growth.
Dig Deeper
Looking to implement generative answering across your organization? In this white paper, we explore key considerations to help you build a solid business case for using this innovative technology.