Online recommendation technology in retail has progressively improved over the past 20 years.
Initially, global popularity algorithms were used to generate recommendations, but only to moderate success. Then came collaborative filtering techniques, which uses a complex stream of historical customer data to make more precise product recommendations.
These new algorithms introduced true machine learning AI technology to the fold. Since its introduction, however, advancements in recommendation technology have been minimal. Customers haven’t seen a major difference in recommendation experiences for many years, and it’s not uncommon for some truly baffling product recommendations to still appear in personal feeds.
However, the next major leap forward for recommendation technology in retail is upon us, which should significantly improve ecommerce: deep learning product recommendations.
A major difference between previous recommendation algorithms and deep learning product recommendations is that the latter showcases innumerably more relevant products and utilities, often in the long tail of the product catalog. Read on to learn how this new technological advancement works and why intelligently ‘wagging the tail’ of your product line can make a big difference.
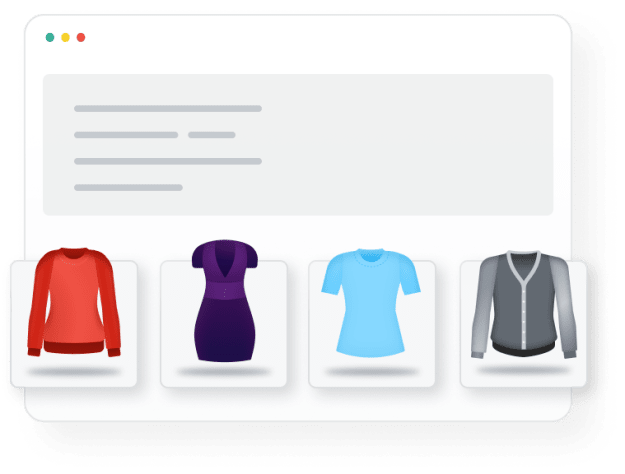
The Limitations of Current Recommendation Engines
It’s important to understand a few of the technological elements and terms involved with this change in recommendation technology.
First, the technology that powers the recommendations lies in the field of Artificial Intelligence (AI). AI encompasses both Machine Learning (ML) and Deep Learning (DL) and relies on the constant flow and parsing of multitudinous data points (such as purchase history, ratings, tagging, metadata, and the trends of other user’s buying history) to give relevant suggestions when users browse an ecommerce website.
The vast majority of current product recommendation tools are based solely on machine learning models, with the most prominent being collaborative filtering.
This approach needs a vast amount of product data to build the product-to-product relationships needed for recommendations to allow for better product discovery. For larger businesses that collect a never-ending torrent of data, like Amazon, this isn’t a problem — but smaller businesses with fast-changing catalogs tend to suffer for a lack of pertinent data sources from product catalogs.
What tends to happen in these scenarios is that the product recommendation system tends to suggest only the top 30% of inventoried products. Our own research shows that these top (aka hero) products are only relevant to 16% of visitors, meaning that your company’s attempt to help your customer and make further sales probably ends right there.
This is especially frustrating when your company has the complementary product they are looking for in the less popular (aka tail) of its inventory.
The Tail End Benefits of Deep Learning
One of the starkest differences between collaborative filtering and deep learning recommendations is the sheer number of product recommendations the latter can offer in a given search result.
Deep learning recommendations show 300x more product suggestions than the current ML-based algorithm. If this information is intelligently filtered, you can provide customers better personalization while simultaneously pushing (and popularizing) long tail items.
Dipping into the long tail of a product catalog helps ecommerce businesses in a number of ways:
- Gives the customer the a highly relevant experience, as evidenced in the 100% increase in click-through rates we have seen in our recent case study with MandM Direct, Europe’s leading online off-price retailer
- Reduces bounce rates since the customer won’t get fatigued or frustrated looking for the right products
- Provides businesses with the opportunity to increase sales of less popular products during non-sale periods, as opposed to during inventory-cleaning clearance sales
Deep learning product recommendation technology is powered by Google Cloud, and utilizes not just their engineering but their best practices. It’s important to note that Google owns YouTube and employs one of the best recommendation systems on the web, with over 70% of video views fueled by their recommendation engine.
This ability to find relevance can be game-changing for businesses of any size.
At Coveo-Qubit we are data-driven, enabling us to be laser focused on achieving high success rates for our customers. Generic solutions that treat every customer the same breed generic results.
That’s why we’re always at the forefront of new technologies that enable us to drive a truly personalized ecommerce customer experience. We’re here to make ecommerce better.
If you think your product recommendations are underperforming or you’ve seen them plateau, we invite you to learn more about Coveo’s recommendation engine.
Dig Deeper
Looking to offer better personalized recommendation? Check out our Your Guide to Personalized Product Recommendations in Ecommerce blog post.
And learn the best practices for keeping your product catalog clean by reading How Amazon Stole Ecommerce Search (And Your Product Data, Too).