Imagine searching for a product without knowing what it’s called. You may have seen it in a store, on social media, or even in passing — but how do you find it online? Enter visual search.
Visual search is rapidly evolving and making a significant impact across a variety of industries, with major web search engines like Google and Bing introducing visual search functionalities that allow users to query information using images rather than words. Apple has now joined the trend, integrating advanced visual intelligence into its latest iPhone, further demonstrating the growing importance of this technology.
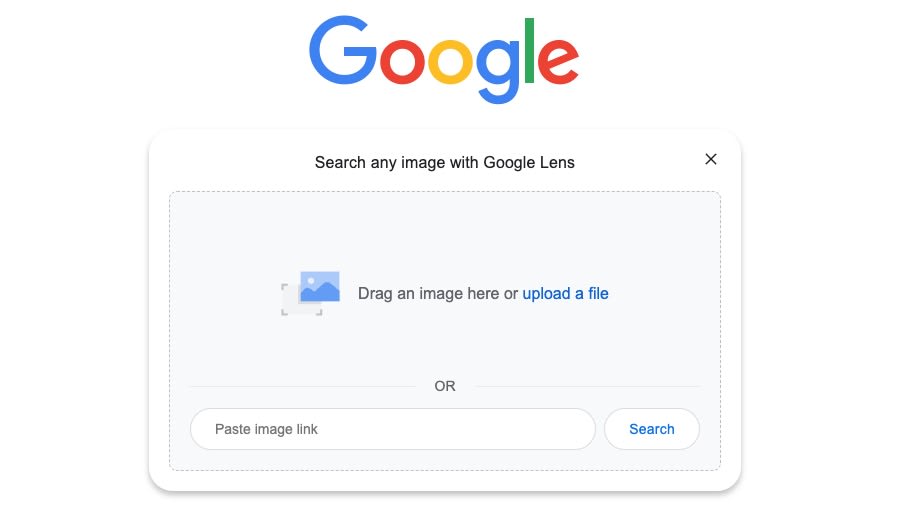
It Started with a Dress
In 2000, Jennifer Lopez wore a now-iconic green jungle-print Versace dress to the Grammy Awards, an outfit so captivating that it sent the internet into a frenzy. At the time, people were eager to find images of J.Lo in that dress, but search engines weren’t equipped to handle the overwhelming demand. This momentous event led Google to create its groundbreaking image search feature, forever changing how we find and interact with visual content online.
In ecommerce, visual search, often referred to as “visual shopping” is rapidly gaining traction. This feature enables customers to search for products simply using an image instead of relying on traditional text-based queries. However, there is a common misconception that the technology underlying visual search is solely focused on searching for products by uploading images. In reality, the technology encompasses much more and analysts like Gartner have expanded the concept, introducing categories such as “visual intelligence” to highlight the broader applications of visual search, which extend well beyond simple image matching.
Visual search technology encompasses a broad set of use cases, including recommendations, vector search, and more. This versatility presents both opportunities and challenges for brands, retailers, and B2B organizations as they experiment with integrating visual search into their customer experience strategies.
Understanding Visual Intelligence
At the core of visual search lies visual intelligence, a technology that leverages real-world images, video, and text to help customers find products with relevant visual attributes. Unlike traditional search, which relies solely on text, visual intelligence expands on visual search capabilities by identifying specific products, providing related content or detailed information, and triggering customer engagement in a variety of ways. This technology is powered by a combination of computer vision, natural language processing (NLP), and machine learning (ML), which work together to analyze product catalogs, understand taxonomy and attributes, and ultimately enhance the customer experience.
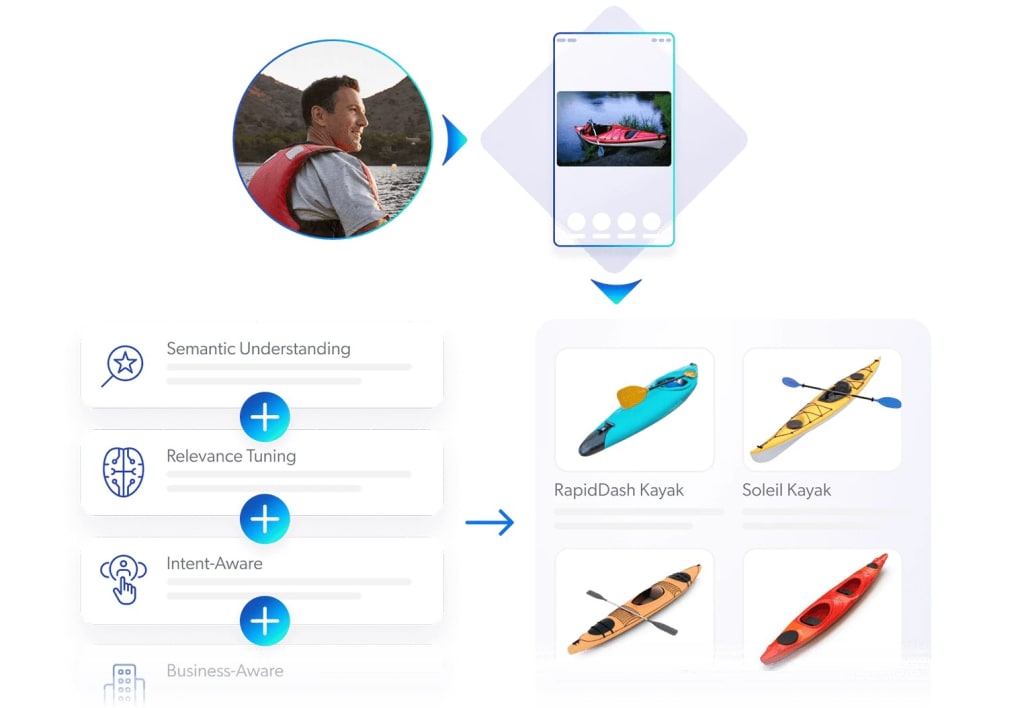
Search by Image
Let’s start with the most well-known use case: image search. While typing into a search box remains the most common way to initiate a search, text-based queries have inherent limitations that can hinder the user experience.
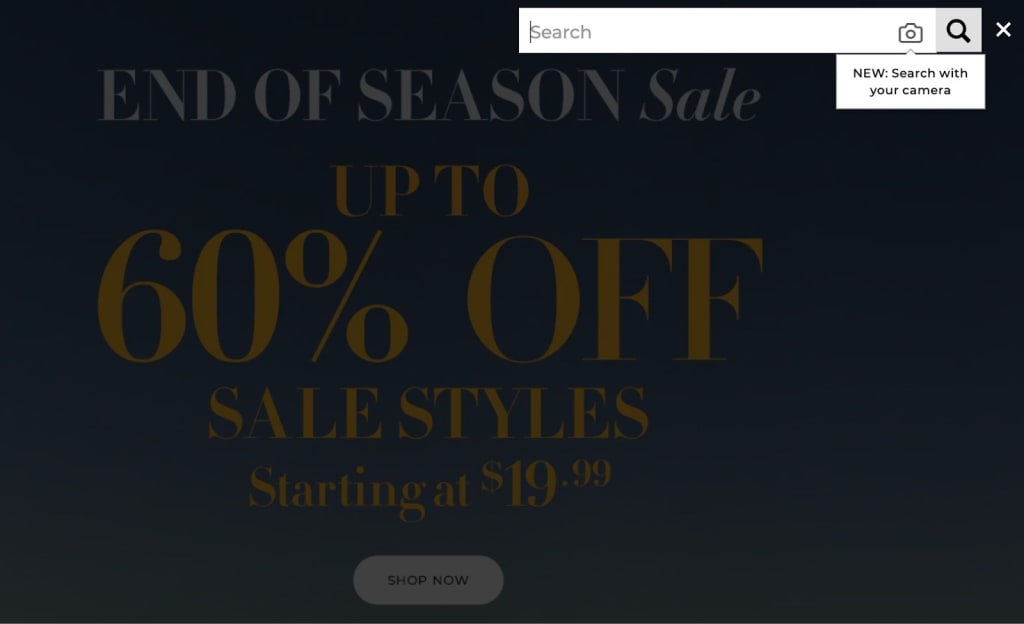
Searching by image offers a valuable solution that alleviates the need for customers to guess the correct names or terms when looking for products. Enabling image-based search functionality can allow for a richer shopping experience. Image recognition capabilities further reduce the friction of searching for items, particularly on small screens and within broad product assortments.
Search by image has several potential advantages over traditional text-based search. First, it can be fast and intuitive, as simple as uploading or taking a picture and triggering a search. Second, it’s language-agnostic, which is increasingly important as online shopping becomes global. Finally, it does not require customers to be familiar with the terminology used by the ecommerce site for the merchandise they are seeking. For example, some users might search for “jeans with holes,” but the relevant products are described as “distressed jeans.” Visual search can bridge this gap.
In categories like fashion, home decor, or art, where products are primarily defined by visual characteristics that are difficult or even impossible to accurately describe by text, visual search becomes particularly powerful. Even after filtering by various attributes, users may still face hundreds of items that differ by style. Visual search technology helps express these aesthetic aspects in a way text has never been able to capture.
In principle, visual search is not limited to B2C use cases; it could also bring significant value to B2B organizations, especially in industrial manufacturing, as suggested by Gartner analysts. By enabling quick identification of parts based on images, visual search can potentially improve efficiency and reduce the need for manual data entry. This is particularly useful for customers who need to find replacement parts for equipment or machinery without knowing the exact serial number or product information.
Visual Search Challenges: Why Some Images Just Don’t Match
However, there are significant challenges, which explain why the technology hasn’t fully taken off yet. Image search can work very well but also faces several challenges across different verticals:
- Object shape and design: Image search works well with some objects, especially symmetric ones, but is less effective with others.
- Object orientation: The same object can yield different results depending on its orientation, such as right-to-left or left-to-right.
- Object background: Issues can arise when images contain significant background clutter, with the object taking up only a small portion of the frame. Confusing backgrounds can also hinder image search.
- Illumination: The same object presented under different lighting conditions can lead to varying results.
Besides these technical challenges, there are conceptual limitations as well. Image recognition technology works exceptionally well in fashion and apparel, where the physical appearance of a product is often the most critical aspect. However, in cases where the physical appearance is less critical and design or internal features matter more (such as with tools or machinery), visual search may not be as effective.
Additionally, implementing and maintaining the technology comes with significant challenges. One of the primary obstacles is the diversity and quality of images in a catalog, which introduces noisy data that can degrade the accuracy of visual search algorithms.
Unsurprisingly, the click-through rate and average number of clicks are substantially lower for image queries than for text queries. While this is not uncommon for a new query modality, it also suggests that there is considerable room for improvement in serving image queries as visual search is still in its early stages.
Other Visual Intelligence Use Cases
Visual intelligence can be applied in a variety of ways, each offering unique benefits and addressing different aspects of the customer journey:
- Image to Text: AI’s understanding of images is helpful but new and imperfect. This use case involves providing short, AI-powered descriptions of an image. Upload an image, and the tool will describe it. For example, Coveo researchers released a model for the fashion domain that supports this use case, outperforming OpenAI’s CLIP model! This capability can support deep tagging – the process of creating and assigning tags to inventory items to simplify and enhance product attribution. While this used to be a manual process, visual intelligence can automatically identify features from images using computer vision and machine learning, adding detailed product tags with vertical-specific lexicons and labels. These rich product attributes are then used to describe items, show relevant search results, create categories, and structure website navigation.
- Catalog Enrichment with Product Vectors: Visual intelligence isn’t just about finding products – it can also enhance product catalogs by enriching them with detailed attributes and image metadata. In ecommerce settings, where bounce rates are typically high and recurring users are rare, in-session personalization is key but requires highly granular representations of a catalog. For example, Coveo researchers illustrated how they could achieve that by leveraging images, improving vector search capabilities.
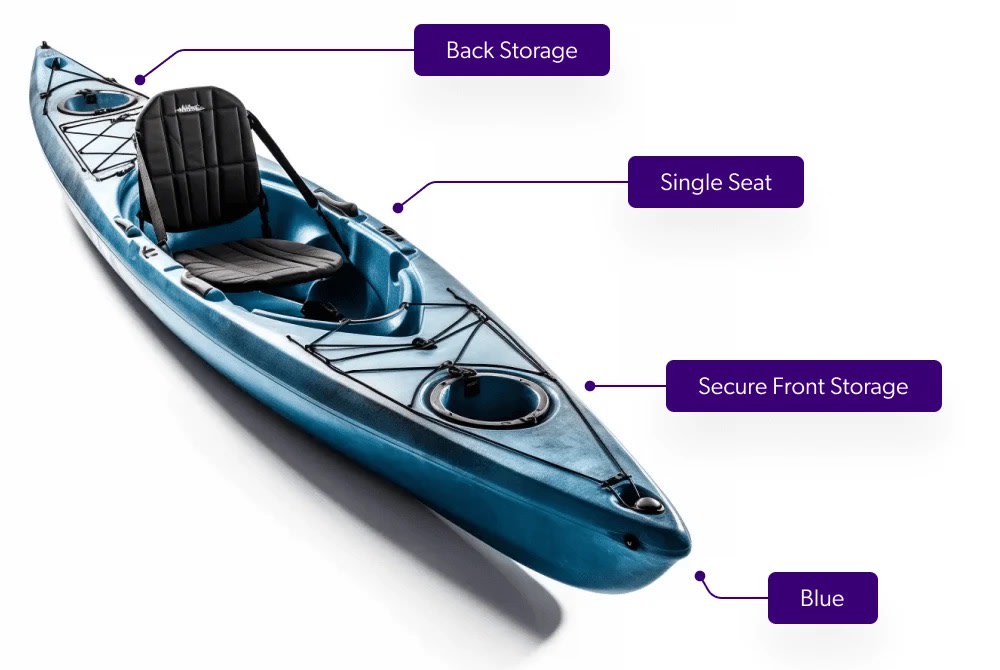
- Visual Recommendations: Visual search technology can deliver great value to customers and businesses even when searches start with a text query. Visual recommendations allow ecommerce players to recommend relevant products to shoppers based on similarity in patterns, style, color, or shape. But visual intelligence can be used to power other use cases for recommendations. For example, Coveo researchers introduced a new type of recommender system in the context of ecommerce, called gradient recommendations.
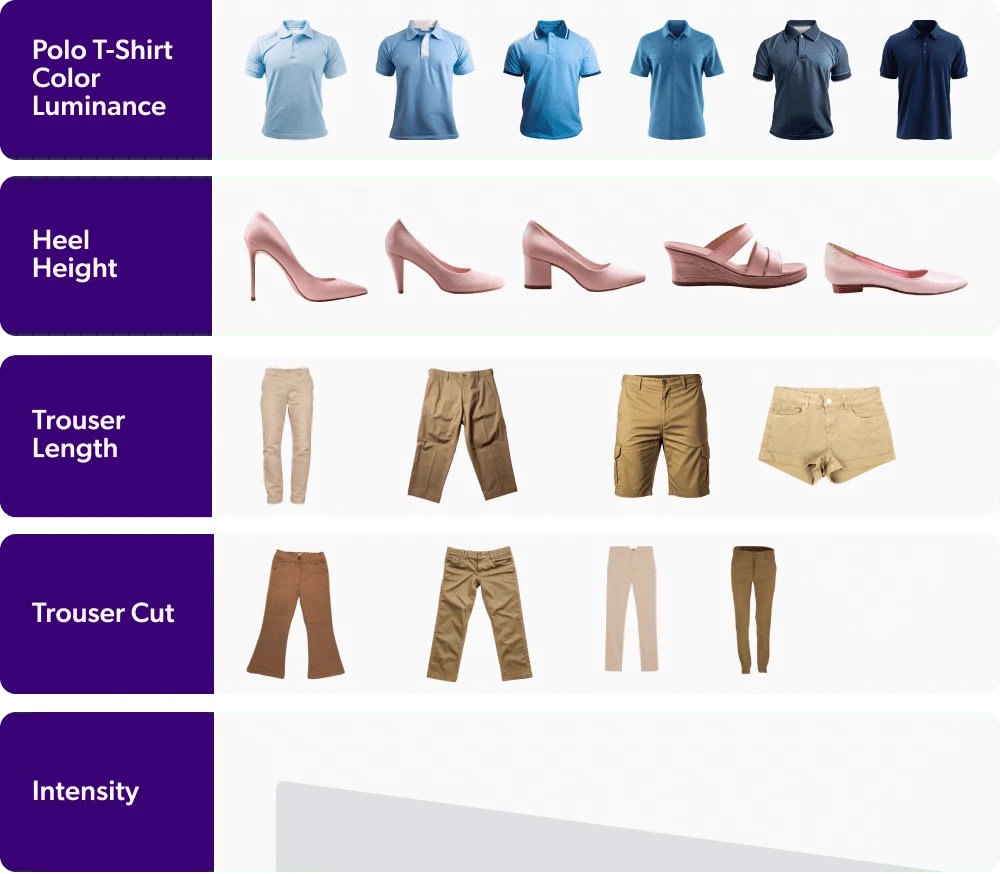
These recommendations suggest products closely related to an item under consideration but with varying attributes such as color or heel height. This mimics real-life shopping experiences where shoppers ask for similar but slightly different products.
The Future of Visual Search
As visual search continues to evolve, its potential to play a transformative role in ecommerce is becoming increasingly evident. However, it’s also clear that visual search is not a one-size-fits-all solution.
Brands, retailers and B2B organizations need to carefully consider their specific needs, potential challenges, and choose partners that can deliver the best results for their customers. At Coveo, we’re continuously exploring and testing new technologies to stay at the forefront of innovation and our commitment to research and development is evident in our recent studies and experiments. If visual search is a relevant use case, we can integrate with third-party solutions specialized in visual intelligence and help you choose the right partners. By integrating visual search with other technologies, brands and retailers can unlock its full potential and deliver exceptional customer experiences.