The biggest danger that generative AI represents to organizations is overinvestment followed by disappointment. The drawbacks that lead to that danger are noted everywhere, most especially in hallucinations, where the AI gives (often) plausible sounding responses that are not correct.
As NPR’s Planet Money put it, “The artificial intelligence we have now isn’t actually that intelligent.” That’s because chatbots based on public or open-source large language models (LLMs) aggregate information from the internet, but they don’t have any way to tell if the information is correct or to reason.
Fortunately, Coveo helps solve that issue by creating a feedback loop that feeds LLMs with your data and with data gathered from human interactions on your site or intranet. The result is that users get spectacularly relevant information. How? AI alone isn’t enough. The real differentiator is relevance — the ability to deliver context-aware, predictive, and highly personalized digital experiences at scale.
In other words, Coveo provides a means to make every website interaction fit exactly the person using the site, whether in customer service, ecommerce, or the workplace.
For enterprises that can realize the potential of AI that ChatGPT and other generative AI products offer — by providing contextually accurate responses —the advantage is profound.
At a recent Relevance 360 event, Coveo’s Executive Chairman Louis Têtu put it bluntly:
“Digital is table stakes, but relevance is not. Every enterprise delivers millions of interactions every day. The ability to make each one relevant is the competitive edge that separates market leaders from the rest.”
The Competitive Advantage of AI-Driven Relevance
Organizations that master AI-driven relevance are seeing double-digit improvements in self-service success, customer satisfaction, and conversion rates — while reducing operational costs. Those that don’t risk falling behind in an increasingly AI-driven world.
Our 2025 Customer Experience (CX) Relevance Report found that 72% of customers will go to Google or a competitor if they experience an unsatisfying digital experience.
Têtu captured this shift in user expectations:
“People are saying, ‘Don’t waste my time.’ They’re only a browser window away from getting a better experience somewhere else.”
The cost of that is high. A poorly trained chatbot, a knowledge base filled with outdated content, or an AI model that delivers generic, inaccurate responses can lead to frustration, churn, and inefficiency.

What It Takes to Achieve AI-Driven Relevance
To understand how AI and search deliver relevance, let’s talk about data and take a brief look at the tech that got us here.
Data is everything, everywhere, all at once. This data bounty makes LLMs possible, but it also means your apps have quite the job of sifting through it all to find what you, your customers, and your employees need.
Solving that search issue is a matter of boosting your AI with your organization’s data, creating a feedback loop that continues to improve results. The more you use it, the better it gets.
To move beyond basic AI implementations and deliver highly personalized digital experiences, enterprises need to get four things right:
1. Unified Data: Breaking Down Silos to Provide Context
AI can’t generate relevant answers if it doesn’t have access to the right – or all – data.
Most enterprises store knowledge across repositories. For example, the Technology and Service Industry Association found that, on average, support relies on 17 knowledge management repositories on any given day to conduct their work. And that doesn’t include employees who also need to access and use data from CMS platforms, ticketing systems, product documentation, and more.
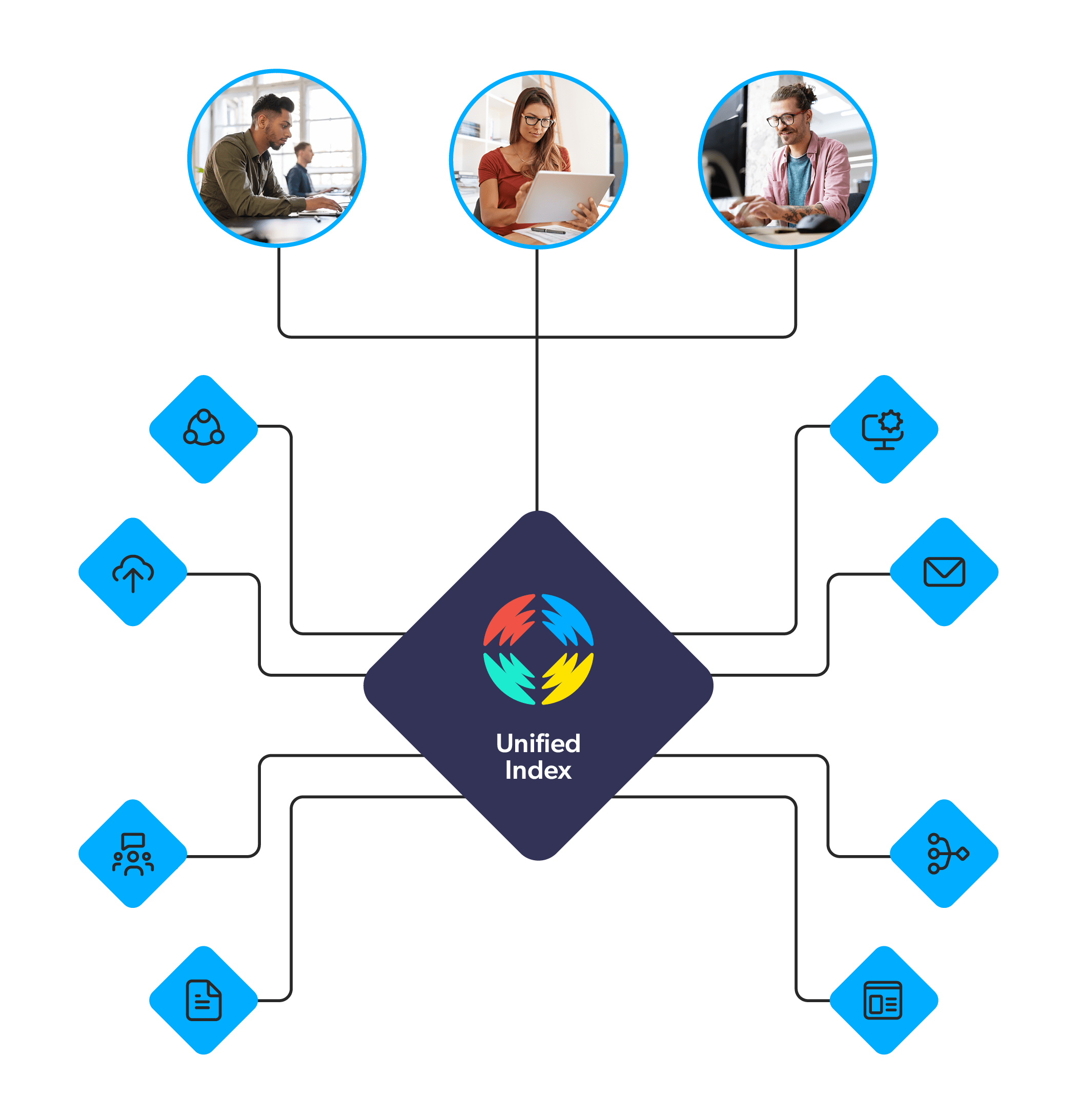
Without unified indexing and secure, real-time retrieval, AI struggles to deliver accurate, up-to-date responses. Many technologists believe that looking across all of this data means normalizing it to put it into a common repository or creating complex queries to join tables, both of which cause time delays and data sync issues. And, perhaps, some black magic, because those queries are complex enough to get saved and put into their own repositories. Coveo addresses this by having a unified index – leaving the data in its original eplace while being able to ask questions of it.
Relevant reading: Why Unified Search Is a Must-Have for Generative AI
2. Personalized Context: Understanding Users at an Individual Level
Relevance is not one data point; it’s the ability to combine multiple aspects that contribute to a fuller picture of a need or ask in that current moment. It requires understanding multiple facets: intent, preferences, and historical interactions. Traditional persona-based approaches group users according to an idea of something they have in common, and treat all grouped users the same, rather than adapting to each individual.
AI-driven relevance automatically adjusts content, recommendations, and responses based on real-time user signals by looking at what the user is doing within a session, akin to how a store clerk might observe a person in a store—picking up one thing, putting it down, choosing another. In this scenario, it’s clicks, page visits, and other relevant, timely information.
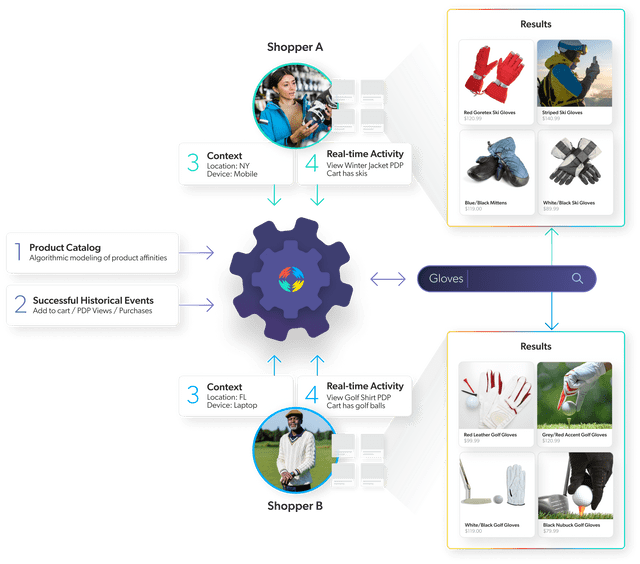
This works even if you have no historical data to draw from, because you can start customizing and personalizing the experience with the user’s first click.
Relevance results from a dynamic process: following the user, collecting data dust along the way, and feeding it to machine learning models so that it adapts as the user does. It studies all previous searches for a specific term and analyzes whether different pieces of content were more or less satisfying to the people who did the search.
Relevant reading: Generative AI and other top AI models for Customer Experience
3. Search-Augmented Generative AI: The Key to Accuracy and Trust
Combining LLMs and traditional search techniques is retrieval augmented generation (RAG). Google, Amazon, Nvidia, to name a few, talk about the importance of RAG in providing more accurate information to users.
Têtu also emphasized the importance of RAG:
“Generative AI without relevance is a liability. It needs to be grounded in real sources of truth—secure, traceable, and constantly refreshed.”
For example, Dell integrates search, GenAI, and recommendations into a single unified system. If a user searches for “Alienware laptop overheating,” they receive:
- A curated list of relevant support articles
- A generative AI response summarizing troubleshooting steps
- Product recommendations if replacement parts are needed
Rather than a list of unrelated results that they have to wade through to find anything remotely related to their search.
This unified experience ensures that AI responses are accurate, trustworthy, and useful—driving higher self-service adoption and reducing support costs.

Keyword Intent: Matching What was Said to What was Meant
But how? More often than not, a search algorithm focuses on what the content is about, not necessarily on matching what the searcher is asking with the most relevant result.
That’s because search technologies break down the words used in documents to determine a relevance score or quality score. But those methods don’t take into account the searcher’s intent — and true search relevance is all about how well a search result meets a specific need.
For example, a search query for “alien movie” returns results on the Alien movie and franchise, movies about aliens, articles about the actors, producers, and writers of said movies, where to stream those movies, and so on.
In the past, search technologies used a ranking algorithm to calculate a relevance score by looking at the frequency of keywords within a relevant document. But that scoring model doesn’t distinguish between the movie named Alien and movies with aliens in them. Nor do they show you what you may want to know about aliens in movies. In other words, we don’t know much about the searcher’s intent from the keyword alone.
The issue around search relevancy has been around since humans started archiving information, and it’s been a matter of research since computers could store information.
Retrieving More Than Information
Information retrieval methods use natural language techniques of vectorization (a way of turning textual data into configurations of numbers that machine learning algorithms can then process and ultimately find patterns in), indexing (making a list of where every word or phrase can be found in a set of text), and term frequency (how often a word appears in a document helps determine what that document is about) to break down text.
Ranking algorithms on the web help determine what documents are more closely related to others and tackle the problem of relevancy by using web page links as a proxy for topical relevance. Enterprise search has relied on manual processes for ranking that involve:
- Search schemas that control how and what people use to search. This, of course, limits what searchers can find and relies on everyone to think along similar lines.
- Query rules return specific results based on a query. For example, sites can use query rules to promote sales products to the top of search results for a specific promotion.
- Synonym matching that allows users to type one term, and get results that use a different term that means the same thing. Ecommerce sites use this to make sure there are no null results for the users’ query. To ward against this, merchandisers often manually pore over log files looking for misspellings and synonyms. These words and typos are carefully added to a thesaurus or dictionary.
- Persona-based rules create actions based on the user behavior of the person the site thinks is visiting. Writing enough rules to be useful takes a lot of time and requires constant maintenance, and everyone matching that persona gets the same experience.
There are more manual ways to tune search engines that involve poring over search logs for long-tail results, adding wild card search to external and internal sites, autocompletion, and more. These things do boost relevance ranking, but they are onerous, and it’s impossible for humans to keep up with changing language and fashions.
Enter artificial intelligence, triggered by the weariness of both searchers and search managers.
Search-augmented generative AI improves search relevance by automatic synonym detection, ranking algorithms that learn from feedback, and geolocation information, tracking user activity on-page and throughout the site during a session to understand the users’ site search habits – and combining those with LLM results to harness the power of AI to sift through and make sense of this context-specific data.
Relevant reading: What Is User Intent (& How It Improves Conversion Rates)
4. Continuous Optimization: Measuring and Improving AI Performance
Relevance isn’t static. It requires constant learning and refinement based on user behavior and real-world results. Enterprises that test, measure, and optimize their AI-driven experiences see significantly better outcomes.
Têtu reinforced this point:
“Never believe AI and GenAI claims. There are a lot of those right now. Test and measure on your own data. The benefits are real — but only if you deploy AI that actually works at scale.”
Organizations that embrace A/B testing, analytics, and iterative improvements will maintain a competitive edge as AI technology evolves.
Customers get excited when search vendors promise the ability to effortlessly deploy GenAI that delivers true relevance. Their expectations are that manual work will disappear while the search gets better. Disillusionment invariably follows when they have to continue to manually tune, or when they can’t scale with more product or more documents, or when they do A/B testing and find there is nothing relevant at all!
AI doesn’t need rules to be tweaked—it adapts as it consumes more data, adjusting for who you are and what you like, and what has worked for other people like you in the past.
But there’s more to relevance than search. With search, you can’t be helpful until someone experiences a need or pain and asks for help. With Coveo, you’re not waiting for someone to experience a pain point or have a question. You’re getting ahead of it, predicting what they might need even if the person doesn’t know yet that they need it.
Machine learning again uses everything that a person does online to infer intent. This signal data includes what a person types in a query, what they retype, and what they eventually click.
Signal data can be historic or real-time. Historic data trains the machine on what others have done. Real-time data informs the machine of what the person is doing within a session. Both give signals about what a person wants, as well as what they may need in the future.
How the Coveo AI-Relevance Platform Powers Enterprise-Grade Relevance
Coveo’s AI-Relevance Platform is designed to deliver search-augmented generative AI, hyperpersonalization, and unified knowledge experiences across service, commerce, websites, and the workplace.
Here’s how it enables enterprises to achieve AI-driven relevance:
- Unified Indexing: Connects structured and unstructured data across all repositories.
- Hyperpersonalization: Adapts responses and recommendations to each user’s unique needs.
- Search-Augmented Generative AI: Ensures generative responses are grounded in real, trustworthy data.
- Continuous Optimization: Uses analytics to measure performance and improve over time.
Organizations using Coveo’s relevance technology have seen double-digit cost reductions, revenue growth, and CX improvements. For example, Zoom implemented AI-powered relevance to optimize customer support. The result?
- 19% reduction in support cases
- 20% increase in self-service success
- Fewer escalations, lower costs, and improved CX
These gains weren’t driven by AI alone, but by AI designed for relevance — an approach that anticipates user needs, delivers accurate responses, and continuously improves based on real-world usage.
Relevant reading: How Zoom Achieved a 2.3x Increase in Case Deflection
Final Takeaways – The Future of Digital Experiences is Relevance-First
The enterprises that succeed in the AI era will be those that prioritize relevance over raw AI capabilities. Simply deploying AI isn’t enough — it must be designed to deliver highly individualized, context-aware experiences that optimize business outcomes.
As Têtu describes it, this is a binary competitive advantage:
“Companies that compete against relevance-driven AI will lose. The quantum leap in performance is undeniable — lower costs, higher revenue, and dramatically better digital experiences.”
For enterprises looking to accelerate their AI journey, Coveo offers a proven, scalable approach to AI relevance. Through unifying knowledge, personalizing interactions, and ensuring GenAI is built on a strong foundation of search and relevance, Coveo helps organizations achieve immediate impact — without significantly lowered risk.
Ready to see how AI relevance can transform your business? Explore Coveo’s AI-Relevance Platform and discover how leading enterprises are setting the standard for next-generation digital experiences.
Dig Deeper
Watch the full R360 recording, Real Customer-First Experiences Start with AI-Relevance, now available on demand: