How do you choose the best search solution for your ecommerce platform?
When it comes to choosing a search and product discovery solution for an ecommerce platform, evaluating your options can feel overwhelming, especially with so many vendors touting comprehensive AI capabilities. How do you cut through the noise to find the right fit?
To make this critical decision easier, ask yourself — and your potential vendors — these six essential questions. They’ll help you identify the search and product discovery platform that not only meets your needs but also optimizes the customer experience and drives real business impact.
1. Can the Solution Deliver Relevant Results for Every Intent?
Product search is critical to discovering and purchasing items on ecommerce websites. In a typical product discovery scenario, customers start by entering a query on your site and they expect relevant results regardless of how they search.
On mobile devices, they might enter broad and generic queries like “smart TV,” while on desktop, they might use more complex queries like “LG smart TV OLED,” expecting your site to deliver relevant results using advanced semantic search capabilities. Because you can’t predict how customers will interact with your search function, it’s crucial to accommodate all types of queries.
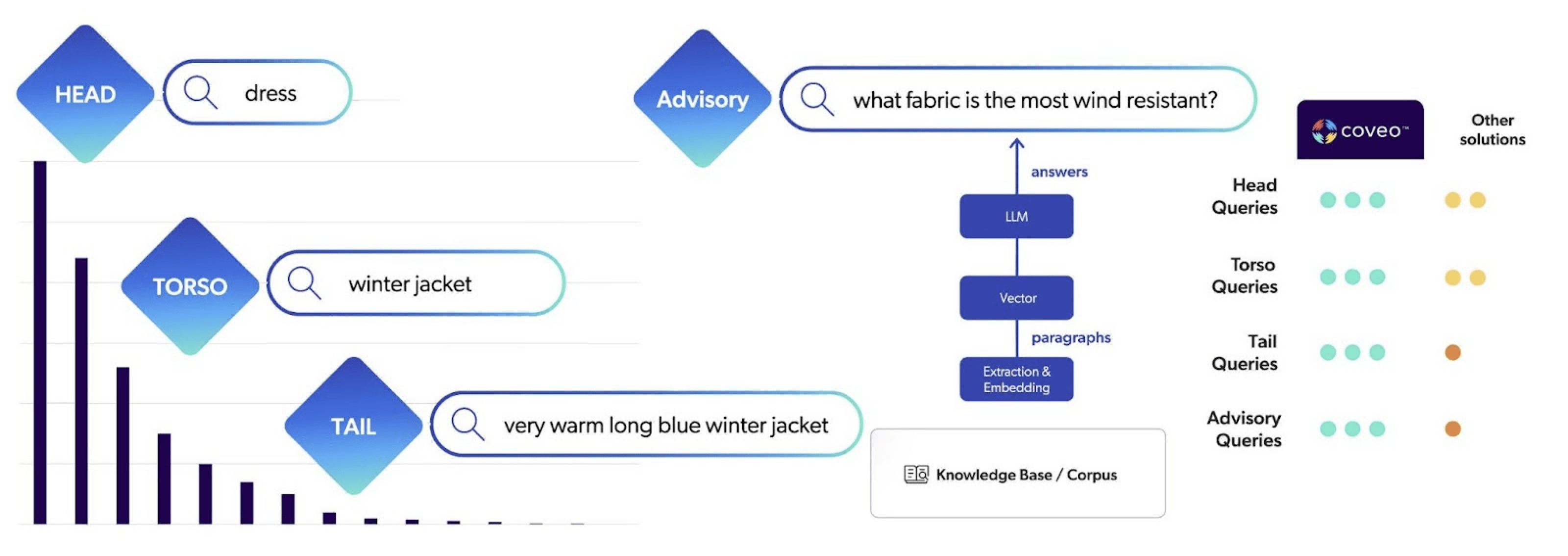
When we look at the typical hockey-stick-shaped distribution of search queries, it’s clear that while a few are very popular, most aren’t. The “short head” represents the small number of high-traffic queries, but it quickly dips into the “long tail” of more niche, less common queries. With the rise of ChatGPT and shifting consumer behaviors, some queries are no longer strictly product-focused but have become more advisory in nature.
The challenge is that most solutions struggle to deliver relevant results for both head and long-tail queries or those that require an answer to be generated. Long-tail searches, which can account for 50% or more of your queries, frequently fail. With so many ways they can miss the mark, it’s impossible for merchandisers to manually curate each query.
Most solutions lack semantic search capabilities that are truly reliable at scale, leaving the long-tail queries largely untapped.
Optimization is needed across the board — not just for long-tail queries, but for all types of searches. While short, high-volume queries might seem easier to handle, most search solutions rely heavily on keyword matching, which often falls short when dealing with synonyms. For instance, a search for “cantaloupe,” “rockmelon,” or “sweet melon” reflects the same intent, but a keyword-matching search engine might miss these connections.
When fat-head queries underperform and return zero results, it’s often easy to identify the issue by reviewing zero-results reports. However, not all fat-head query failures are so obvious. For instance, a search for a popular term like “women’s dress” might return results, but if those results aren’t ranked according to shopper intent, users may still struggle to find what they’re looking for, which might translate into missed revenue per visit.
The traditional search box is evolving into an intent box, where customers explicitly tell you what they need. This shift signals a new era in which understanding intent, not just keywords, is critical. The future of commerce lies in detecting that intent and delivering the most relevant products or answers. By understanding and anticipating customer needs, brands can provide smarter, more intuitive shopping experiences.
Among longer, more complex long tail queries, a new class of conversational queries is emerging that extends beyond the ability to show products. As customer expectations rise with innovations from OpenAI and others, GenAI is transforming how customers learn, discover, and shop. Generative answering can deliver personalized answers rooted in your products and expertise, recreating the in-store experience and positioning your brand as a trusted advisor. Whether it’s answering questions like, “What’s the best lens for wildlife photography?” or providing tips on building an outdoor kitchen with a BBQ, GenAI smooths the path to purchase with helpful, accurate answers.
2. Can the Solution Deliver Relevant Experiences Across Every Touchpoint?
Nailing search is critical, but product discovery goes beyond just search results and is not confined to a single touchpoint. With an expanding range of touchpoints to consider, your customers expect highly relevant, seamless, and consistent experiences across all of them.
For example, consider the importance of query suggestions and relevant filters. Recent Forrester research shows that a significant portion of customers in the US (57%) and the UK (63%) frequently use filters to refine their search results – these numbers are quite impressive.
Yet, the Baymard Institute has conducted extensive research on this topic, revealing that while 80% of ecommerce sites offer query suggestions, only 19% get them right. They also highlight that 34% of websites offer subpar filtering experiences. This finding aligns with our latest research at Coveo, as part of our Commerce Industry Report 2024, which indicates that 35% of shoppers encounter issues with filtering options when shopping online.
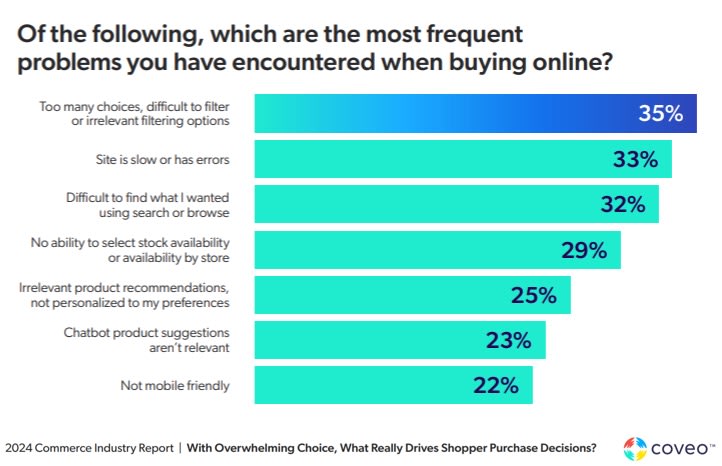
Beyond search, customers increasingly expect relevant recommendations. Until recently, it was common to purchase commerce search and recommendations from separate vendors. However, opting for a single vendor to provide both, allows you to leverage search signals and the same underlying intelligence to improve the customer experience by serving more relevant content throughout their entire journey on your website.
Customers today expect to find appropriate products on listing pages, access rich content, and receive informative answers to their questions. However, all too often, these experiences are underwhelming, leaving customers frustrated and disengaged. The gap between customer expectations and delivery highlights a key challenge for brands and retailers — consistently providing relevant experiences across multiple touchpoints remains a struggle.
Most solutions focus on applying AI models solely to search results, particularly in re-ranking result sets, while overlooking crucial touchpoints like query refinement. It’s essential to ensure your solution can dynamically select the right filters — especially with a broad catalog — and sort them by popularity rather than using static options or applying machine learning to help customers construct more meaningful queries.
3. Can the Solution Deliver Relevant Results for Every Shopper?
Customers expect relevant and personalized experiences. Companies like Amazon have a significant advantage in achieving personalization by leveraging big data tied to user accounts, search history, and interactions with product listings and ads. However most brands and retailers simply don’t enjoy this luxury. Many of their customers are not logged in, are new, or remain anonymous, leaving limited data available to deliver highly personalized results.
This makes it essential to evaluate whether a solution can truly offer personalization for every customer, both known and unknown, by effectively harnessing the signals that shoppers emit to deliver personalized experiences as fast as possible.
Coveo offers true personalization for every customer, both known and unknown, through its proprietary deep learning technology, enabling 1:1 personalization in real-time by leveraging clickstream and in-session data. Unlike some vendors that claim to offer personalization but merely rely on chaotic re-ranking that overreacts to the last products viewed, Coveo’s approach ensures a more thoughtful and relevant experience. So be cautious of solutions that fall short of genuine personalization.
4. Can the Solution Deliver Relevant Results for Every Product?
Cold-start products, (new or niche items with little to no behavioral clickstream data), pose a unique challenge for search and product discovery. As these products have recently been added to the catalog or belong to a niche category, they lack historical customer interactions that would typically inform product ranking strategies. And since most search solutions are relying on behavioral data to inform their re-ranking and recommendations, these solutions are generally unable to deal with long-tail products.
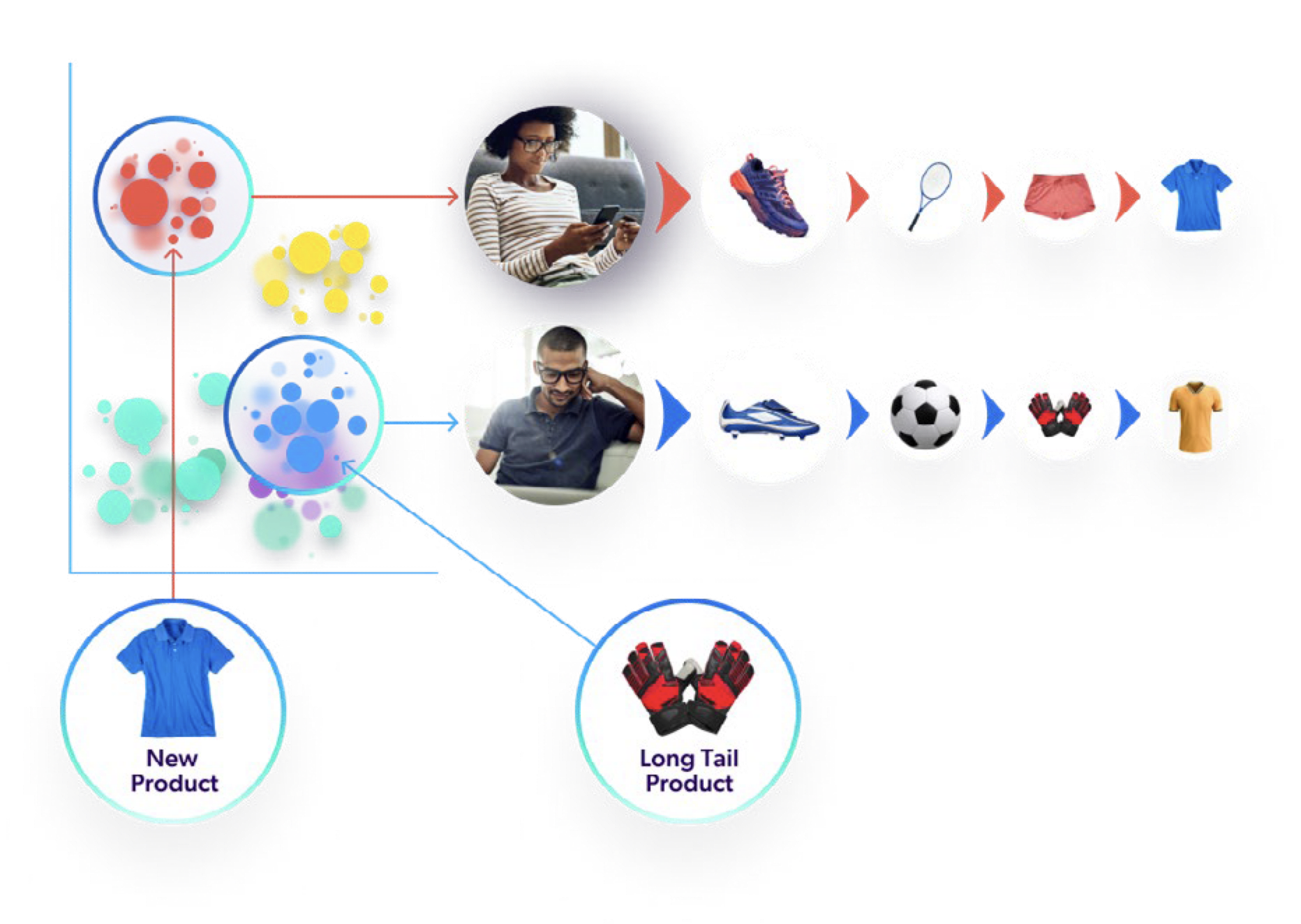
How can you return recommendations for products with no view or purchase data, and support your fast changing catalog? Can you leverage metadata to detect similarities between products, generating relevant recommendations that help shoppers make a decision?
Coveo has invested heavily to build a sophisticated approach to personalizing for new or long-tail products by creating product vectors based on similarities to popular products with higher vector density. This method enhances catalog coverage and enables the re-ranking and recommendation of products with minimal or no prior view or purchase data.
5. Can the Solution Balance Relevance and Ecommerce KPIs?
When choosing a search and product discovery solution, it is imperative to examine whether it can help maximize multiple goals effectively — balancing customer experience as well as ecommerce KPIs.
For your business, search experiences must also be informed by business priorities — driving profit, clearing expiring inventory, and fulfilling supplier commitments. Striking the right balance is critical. If not, the consequences are significant: either business priorities are not met, or conversions drop as customers grow frustrated with a poor experience.
With multiple objectives to consider simultaneously, different interests need to be aligned and balanced. Brands, retailers, manufacturers — essentially anyone selling online — naturally want customers to find products that are relevant and tailored to their intent. However, as a business your priorities likely go beyond just customer satisfaction and presenting the most relevant products during searches.
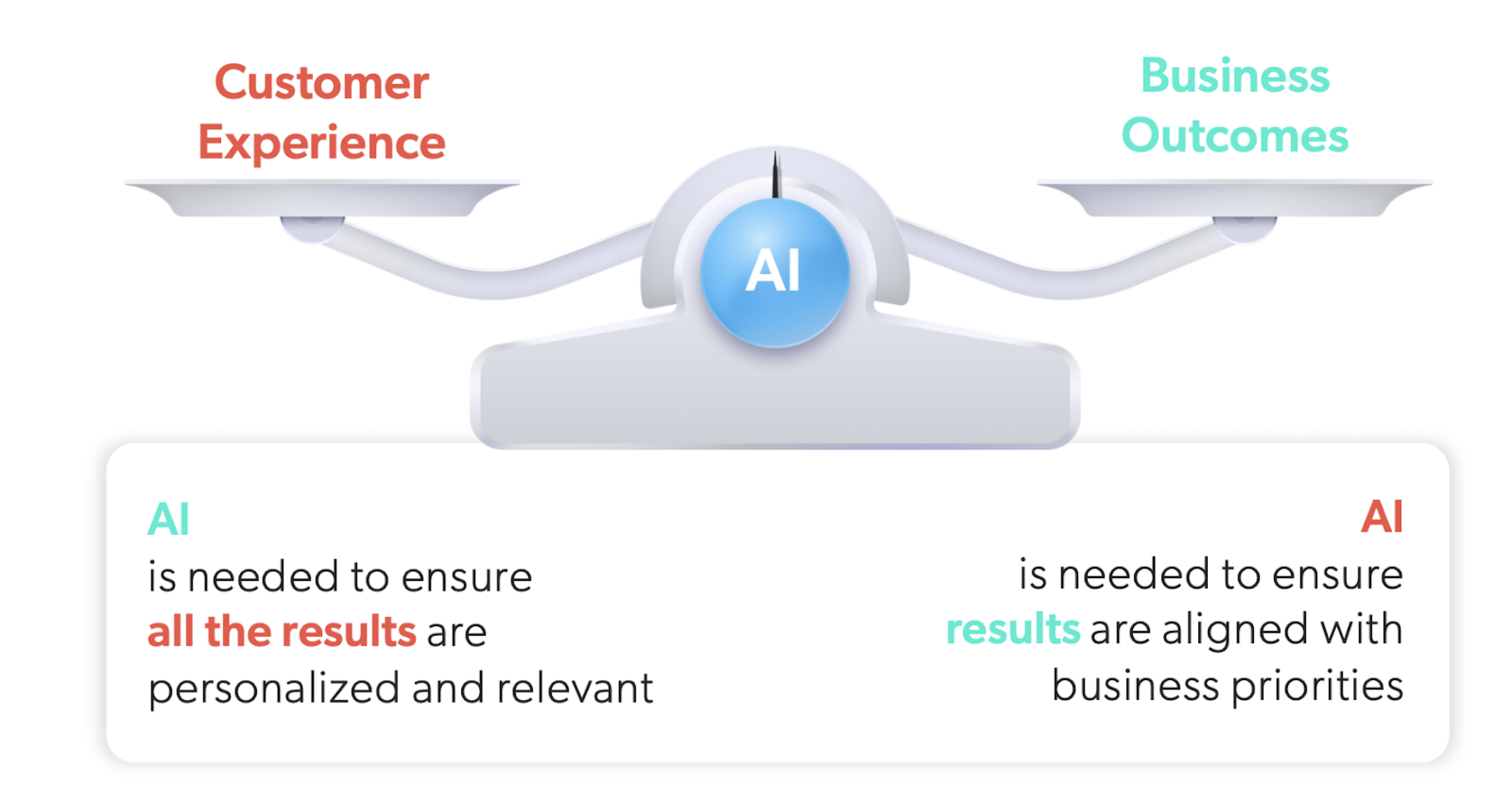
Most search and discovery platforms claim to optimize business outcomes but in reality simply allow merchandisers to apply business rules over their search and recommendation engines. This approach represents a significant missed opportunity, particularly in an era where the value and capabilities of AI are more recognized than ever.
Over-relying on business rules not only limits the full potential of AI to create dynamic, intelligent experiences but can also undermine the fundamental principles of search and recommendation systems. This approach can have a detrimental effect on the customer experience by potentially obscuring items that genuinely interest shoppers thereby lowering conversion and revenues.
Most search and discovery solutions on the market are built on top of technologies such as Solr or Elasticsearch designed for general search applications rather than ecommerce-specific KPIs. These solutions often prioritize metrics like click-through rate (CTR) as a proxy for relevance, assuming that more clicks indicate higher relevance to customers. While this approach might work really well in other use cases, it falls short in ecommerce, where clicks are important, but not always strong indicators of conversions or revenue.
6. Can the Solution Leverage a Wide Range of AI Techniques?
When selecting a search and product discovery solution, it’s essential to evaluate the breadth of AI approaches and tools being leveraged. A comprehensive tool set is necessary to keep pace with technological advancements.
New tools, models, and techniques enable businesses to address a wider range of use cases in search and product discovery with greater accuracy and effectiveness. Additionally, it’s crucial to have the ability to layer models and algorithms, combining them to achieve specific results. For example, you might want to rank results that are both popular and personalized, enhancing relevance for each user.
Generative AI (GenAI) and Large Language Models (LLMs) have recently gained considerable traction, offering innovative ways to enhance customer experiences and streamline operations. Coveo has released critical capabilities that leverage LLMs and GenAI to enhance customer education, enabling them to find answers to complex product-related questions more easily and effectively.
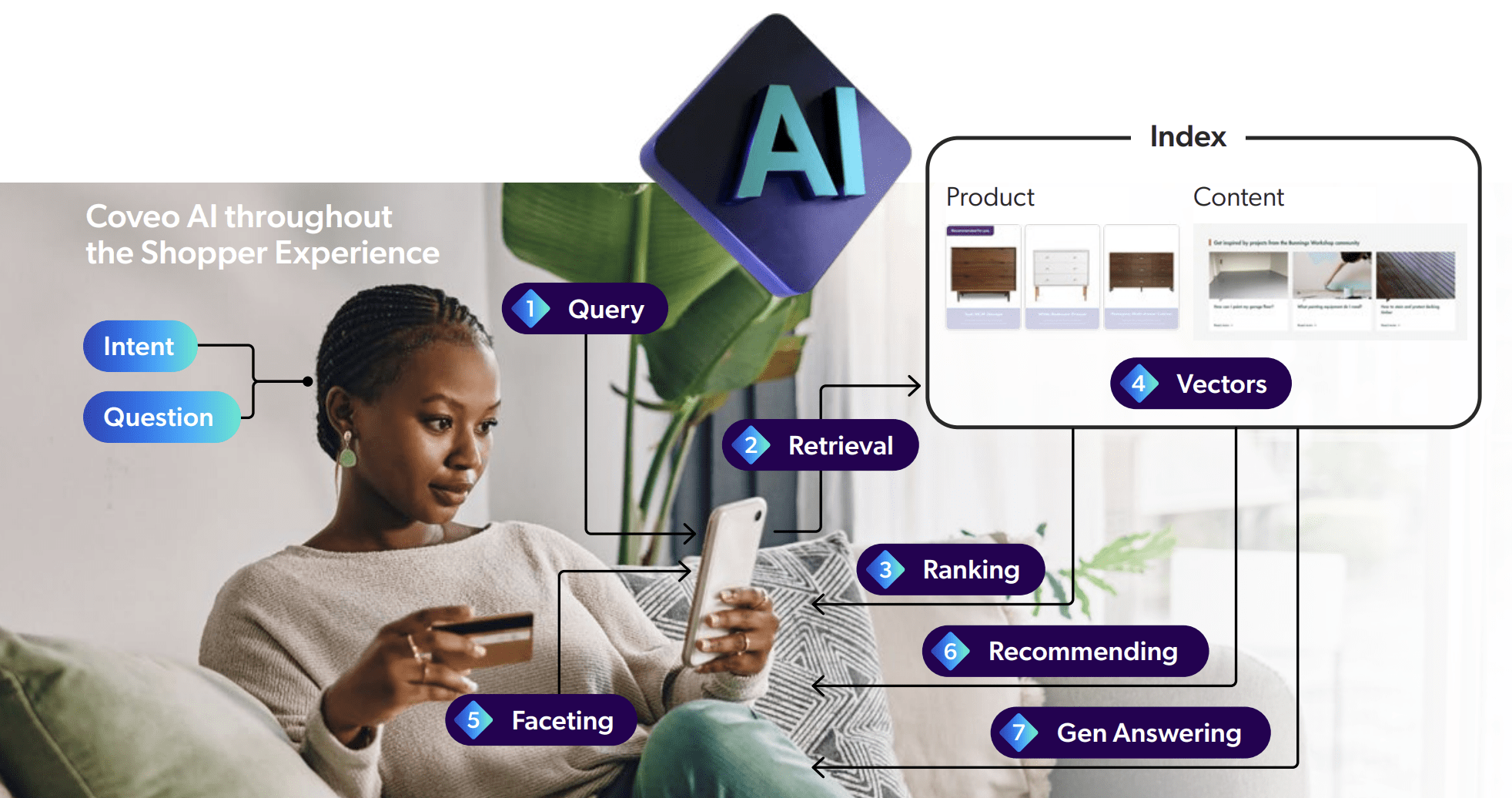
Rather than replacing existing models or methodologies, each technological innovation and approach adds unique value and is best suited for specific applications and use cases. For example, Coveo has been leveraging deep learning models to deliver real-time 1:1 personalization, helping leading enterprises achieve impressive ROI. These advancements complement, rather than replace, the proven strategies already in place.
While there is a prevailing narrative suggesting that the history of technological progress is marked by replacement, the reality is more nuanced. Robust, valuable, and time-tested tools persist. The evolution of AI is a story of complementarity rather than replacement, and it is important to understand which models and approaches vendors are using. At Coveo, we’ve created this guide to help you understand how we leverage them.