Knowledge graphs are a traditional technology resurfacing as an answer to managing the terabytes of structured and unstructured data in the enterprise. And now with GenAI, many are pushing knowledge graphs as a way to make sense of the enterprise; but are they high performance, or high maintenance?
One way knowledge graphs have impacted the digital landscape is by enhancing personalization and recommendations. Netflix, Facebook, and Spotify, as just a few examples, all use knowledge graphs to deliver the recommendations that have helped these companies become the giants they are.
So can knowledge graphs support your enterprise-grade digital experiences? Enterprises interested in offering proactive, tailored experiences might consider this technology as part of their digital strategy.
But maintaining them is costly – requiring staff, resources and budget to get them running effectively. Let’s learn what they are, what they excel at, and their biggest challenges.
Plus we’ll dig into a helpful alternative that can make life easier (while protecting your limited resources).
What is a Knowledge Graph?
Knowledge graphs are a static way to structure and link explicit data signals (such as when a user rates a piece of content as helpful or unhelpful), excelling at organizing information that fits within predefined frameworks.
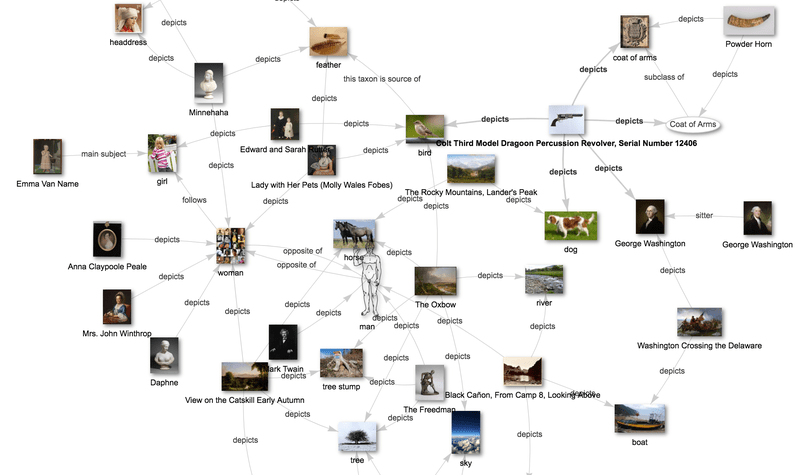
They provide a structured overview of data related to users, products, and content – including the complex interrelationships between them. They organize objects (called “entities” — an actual thing or person, like me, the author of this blog!) and the relationships between them, called edges (like categories, genres, and departments). By mapping out these connections, knowledge graphs help systems understand context and semantics, allowing them to infer nuances.
For instance, a knowledge graph can link product attributes, such as brand, category, and user ratings, with customer profiles, including past purchases and search history, to help offer recommendations and identify sales opportunities.
In a service or workplace use case, this can look like expertise finding. Employees are linked to the docs they produce which are linked to the subjects and then you can have people linked to concepts.
What are the Benefits of Knowledge Graphs?
Knowledge graphs’ structured approach to organizing and linking data is a great advantage for complex information spaces like social media networks.
Let’s explore their key benefits:
1. Streamlining Data Understanding
Knowledge graphs simplify the process of understanding data by bringing together information from various sources into one cohesive view.
They showcase how different pieces of data are connected, making it easier for businesses to analyze and gain valuable insights.
2. Empowering Advanced Analysis
With knowledge graphs, businesses can conduct complex analyses that go beyond what traditional databases can handle.
This includes tasks like asking detailed questions, spotting patterns, and uncovering insights based on how different data points relate to each other. These capabilities enhance decision-making processes.
3. Promoting Shared Understanding
Knowledge graphs foster a shared understanding of data across different systems and datasets. By using predefined structures called ontologies, they ensure that data isn’t just shared but also understood uniformly across applications.
This promotes collaboration and seamless data exchange among businesses.
What are the Challenges of Knowledge Graphs?
Their lengthy development, maintenance, and application can impact their effectiveness and the insights they provide.
Here are some key challenges associated with knowledge graphs:
1. Integrating Varied Data
Knowledge graphs often integrate data from different sources, formats, and domains – which can be a long, manual process. Achieving seamless integration while preserving data semantics requires intricate mapping, alignment, and transformation procedures, which are resource-intensive and prone to errors.
2. Designing and Maintaining an Ontology
Establishing and managing an ontology — a structured framework defining types and relationships within a domain — is fundamental for knowledge graphs.
However, crafting ontologies that accurately capture real-world complexities necessitates extensive feedback from domain experts. Additionally, as domains evolve, ontologies demand regular updates and revisions, adding to the maintenance workload.
While automated updating mechanisms can help, they must be carefully devised to prevent introducing errors or inconsistencies, and human oversight is necessary.
3. Clarifying and Linking Entities
Knowledge graphs must accurately identify and link entities that may have multiple representations across different datasets. Clarifying entities involves distinguishing between those with similar or identical names but different meanings, a process that is crucial for the integrity of the graph but difficult to automate accurately.
4. Extracting and Representing Knowledge
Extracting knowledge from unstructured or semi-structured data sources and representing it in a structured form suitable for inclusion in a knowledge graph is difficult. This process often requires advanced natural language processing and machine learning techniques.
Despite these challenges, knowledge graphs can still be a useful asset for organizing knowledge and enabling complex analyses. Addressing these challenges requires ongoing research, development and maintenance by teams of skilled experts. They also require added attention to improve their data integration, scalability, query efficiency, and privacy safeguards to fully realize their full potential.
What Are Knowledge Graph Alternatives?
An embedding space can serve as an alternative to knowledge graphs for representing relationships between entities. Users are passively providing implicit signals throughout their digital experience journey by interacting with different entities (i.e. content, products, etc.).
A neural network can then be used to generate an embedding space, composed of multiple vectors, to represent these entities and position them closer together if they are interacted with in a similar manner.
These signals include browsing behavior and content engagement, and neural networks analyze these signals to identify patterns, preferences, and behaviors.
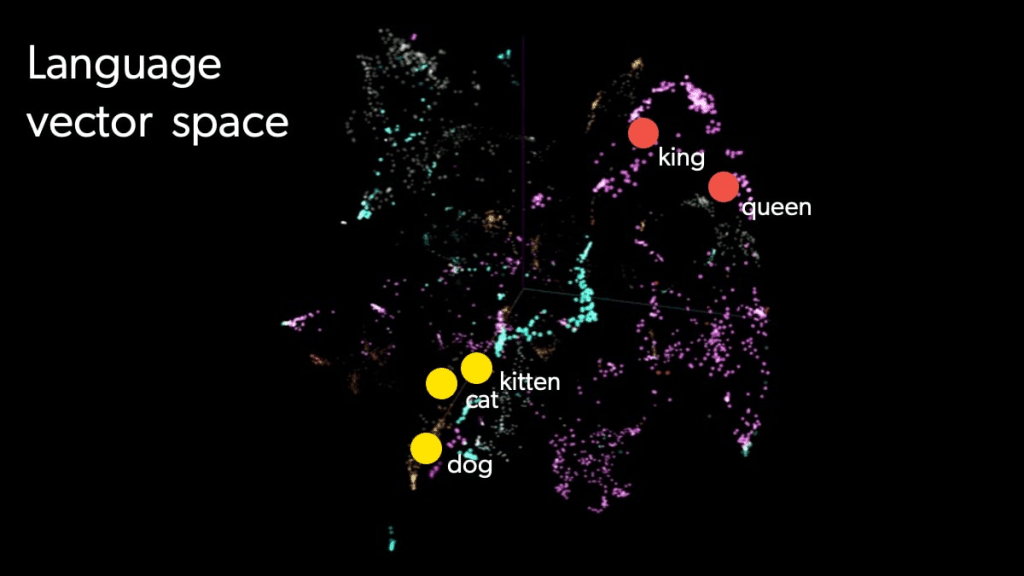
The embedding space can then be used to predict future interactions and enable personalized experiences. And this process improves over time as neural networks are exposed to more signals.
The Coveo Platform™ leverages implicit browsing signals, an item’s metadata and neural networks to build embedding spaces used to personalize and improve query suggestions, search results ranking and recommendations.
What Are The Benefits of Embedding Spaces?
When it comes to customer experience, embedding spaces enhance customer-facing websites by surfacing and recommending the most relevant content based on evolving needs. They can also augment tools like AI-powered intelligent search, generative answering and in-product assistance to help customers self-serve faster.
Recommenders using embedding spaces can enhance the digital employee experience by proactively recommending the right documents needed to get work done. This in turn promotes new employee onboarding and tenured worker upskilling.
Also, embedding spaces can be used to personalize in real-time the user experience in commerce by following the user within the product space.
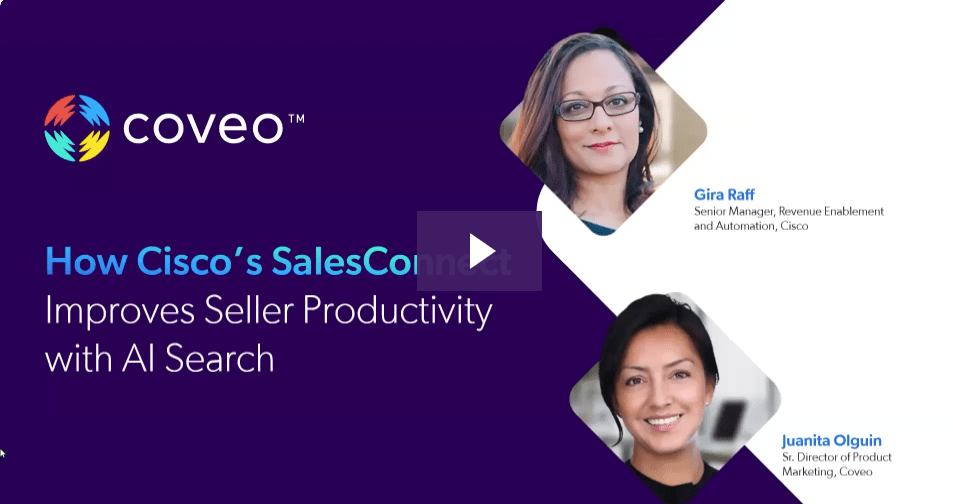
While knowledge graphs provide explicit, interpretable representations, embedding spaces offer efficiency, scalability, and generalization.
Neural networks, used to generate embedding spaces, are exceptionally good at dealing with unstructured, noisy and incomplete data. They’re great at parallel processing, allowing them to process large volumes of data faster and more efficiently.
When provided by the right vendor, they are a powerful, independently evolving and low maintenance alternative to knowledge graphs with higher cost savings.
Embedding Spaces and Knowledge Graphs: Better Together?
If you have your own knowledge graph, Coveo can easily ingest the static snapshot of relationships. With this, Coveo can surface content related to the entities outlined by the graph.
This can also help Coveo further understand the strength of these relationships by feeding knowledge graph data into its continuously improving and evolving neural networks, for maximum pattern recognition.
However, neural networks are already efficient at using unstructured data, so this might be overkill for enterprises with limited time, budget and resources.
When it comes to delivering personalization across your digital experiences, the choice is yours:
Do you have the time, resources, energy, and manpower to create a reliable, structured and static knowledge graph you’ll need to regularly maintain? Or are you able to pay the expensive price of asking someone else to do it for you (and maintain the knowledge graph for the years to come)?
Or does your enterprise need an adaptive, scalable solution that can evolve with your growing needs (and that you can set and forget)?
Dig Deeper
Connect employees with the knowledge they need, without an army of data scientists. See how Coveo’s GenAI overhauls knowledge management by seamlessly integrating with major workplace platforms.
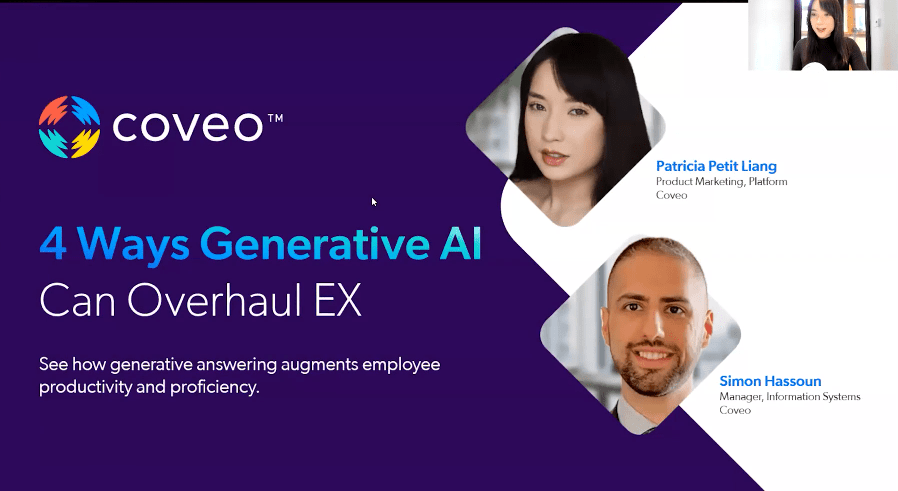