ChatGPT and similar generative artificial intelligence (AI) tools like Bard are examples of natural language processing (NLP) models that have gone mainstream. According to Google Trends, searches for the term “generative AI” have increased rapidly since the introduction of ChatGPT in late November 2022. The chart below demonstrates a popularity index of 100 for the term as of April 16, 2023, up from 0-3 in April through August of 2022.
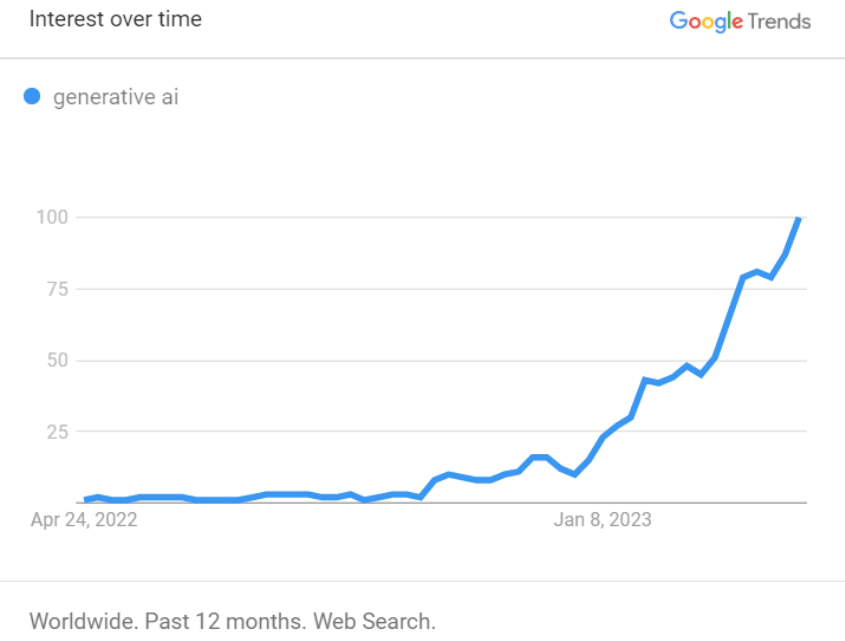
These tools, while powerful, aren’t plug and play. They require massive amounts of data to train and no small amount of effort to use effectively.
Some concerns around generative AI include “hallucinations“—when the AI generated content looks good, but is ultimately nonsensical or the model makes things up, the issue of multiple biases, limits to what the AI knows, and the inability for an AI system to reason. Before embracing generative AI, organizations need to understand what it is and how it works, so they’re prepared to effectively use it.
What Is Generative AI Technology?
Generative AI is a large language model (LLM) that is trained to understand the structure of text-enabling natural language inputs to generate original content. It uses big data sets and machine learning algorithms to generate original text and fluent conversations.
Some generative AI tools employ generative adversarial networks (GANs) — a type of deep learning technology — to create new content. GANs use a deep neural network framework. They create content that shares the characteristics of the data they’re trained on. GANs use a second neural network called a discriminator that’s trained to identify fake data created by the GAN generator. Currently, GANs are used primarily for image generation, for example, Microsoft’s CoModGan uses this technology to complete images that are missing large amounts of information.
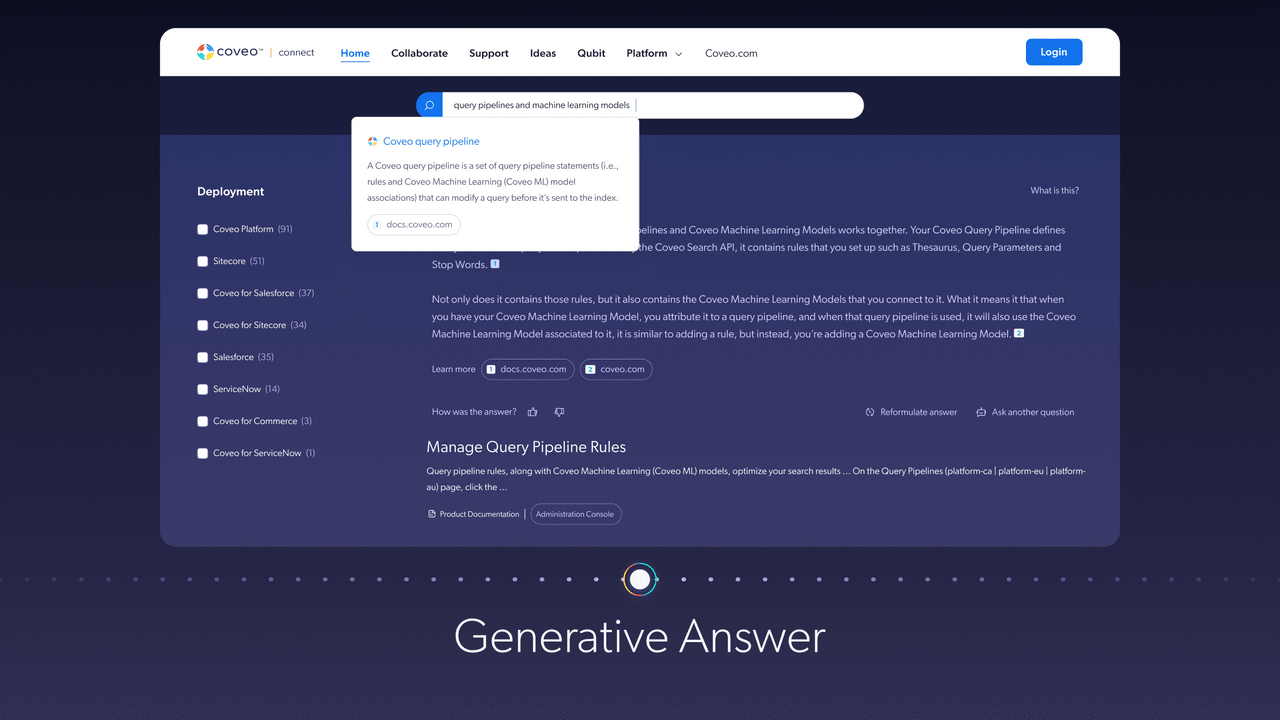
When it comes to text-based content, Generative AI is exciting for many reasons, but particularly for its ability to generate a structured human-sounding response to a natural language query. The implications for building a knowledge base using a generative AI model are huge.
“The ability to engage in knowledge exchange — to ask questions and get ‘the’ answer versus a list of answers — is probably the most intriguing initial use case of generative AI for service leaders,” said Tom Sweeney, Founder and CEO of ServiceXRG, a customer service research consultancy.
What Are Obstacles to Adopting Generative AI?
While the ability to engage in knowledge exchange to ask questions and get a precise answer — versus a list of answers — is promising, there are some barriers to adopting generative AI algorithms and systems into existing workflows. These include:
- Implementation: While the potential to reduce repetitive tasks, create new content, and harness existing data is promising, implementation of generative AI tools can be challenging. Generative AI applications aren’t easily accessible to companies who don’t have the data analysts, engineers, and machine learning experts to define and integrate their content into new language models.
- Effort: Adopting generative AI involves more than just indexing, creating, and adding documents into the new model. It requires a lot of effort to train a generative model to your specific business domain. Effective models also require continuous feedback, sufficient training data, and supervised learning. That is, to be useful they must be able to self-optimize based on a constant input of new data and information.
- Cost: It takes money to create a personalized AI model tuned to your specific knowledge base, product catalog, language, and brand voice. According to MosaicML, a platform that enables users to train large AI models on their data, it costs about 450K to train a model that reaches GPT-3 quality. But cost varies depending on factors like the size of the model, the amount of data used, and the infrastructure used for training.
Despite these challenges, the potential of generative AI use cases for businesses is enormous.
What Are Real World Applications for Generative AI?
The promise of generative AI for your organization lies in the specific use case (or cases) you’ll develop. There are use cases for creating knowledge, for providing answers, and for and using it from a classification perspective.
“This is the first time that we have an tool that can understand the unstructured world,” said Sweeney. “We’re so used to using databases to pull data and run reports and now we have the classification, the extraction of passages, and the formulation of ‘the answer’ in response to a customer question. These are big, profound, and sweeping changes to how the support paradigm is going to work.”
Coveo has been implementing LLMs since 2020 for several use cases including smart snippets and case classification. Smart Snippets extract answers out of document when a user conducts an online search. Case Classification uses machine learning to suggest classification values to end users as they create a new support ticket.
“With smart snippets, those answers are returned as a snippet,” said Kostecki. “You’re probably familiar with seeing that kind of experience in Google and other search engines. We also use LLM to classify information based on previous cases. This automatically classifies some of the field values and helps remove some of the effort that’s required of a customer as they’re submitting a case to support.”
Getting Started With Generative AI
There are four actions that organizations can take when it comes to working with generative AI.
1. Choose a use case
Deciding on a use case and then justifying it is absolutely step one. This sets the stage for everything that comes next — including the type of content you’ll build as part of your knowledge base and how generative AI helps amplify your knowledge strategy.
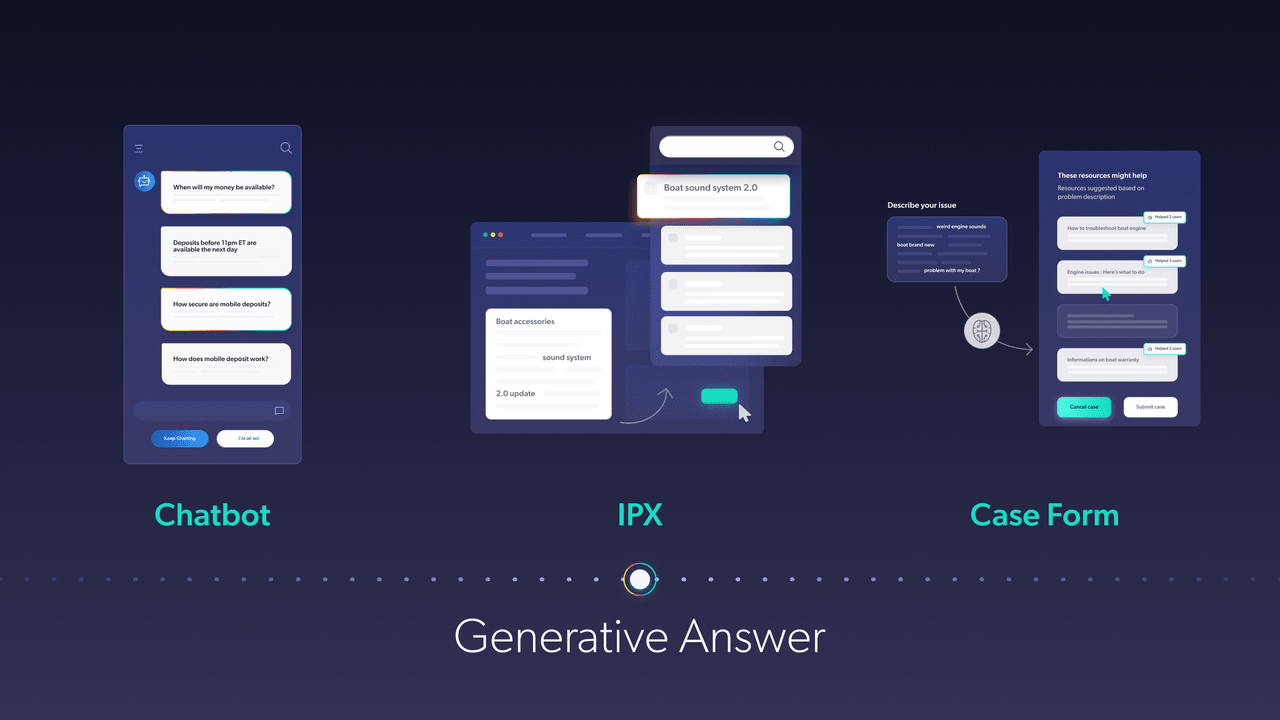
2. Amplify knowledge management
Knowledge is the foundation of Knowledge Centered Service® (KCS)*. Your knowledge program forms the basis of your answers generated by the LLM. Hire knowledge experts that are obsessed with understanding how to solve the problem. They should be able to break issues down and help you establish a knowledge sharing culture focused on collaborative expertise and creating content a technology generative AI can leverage.
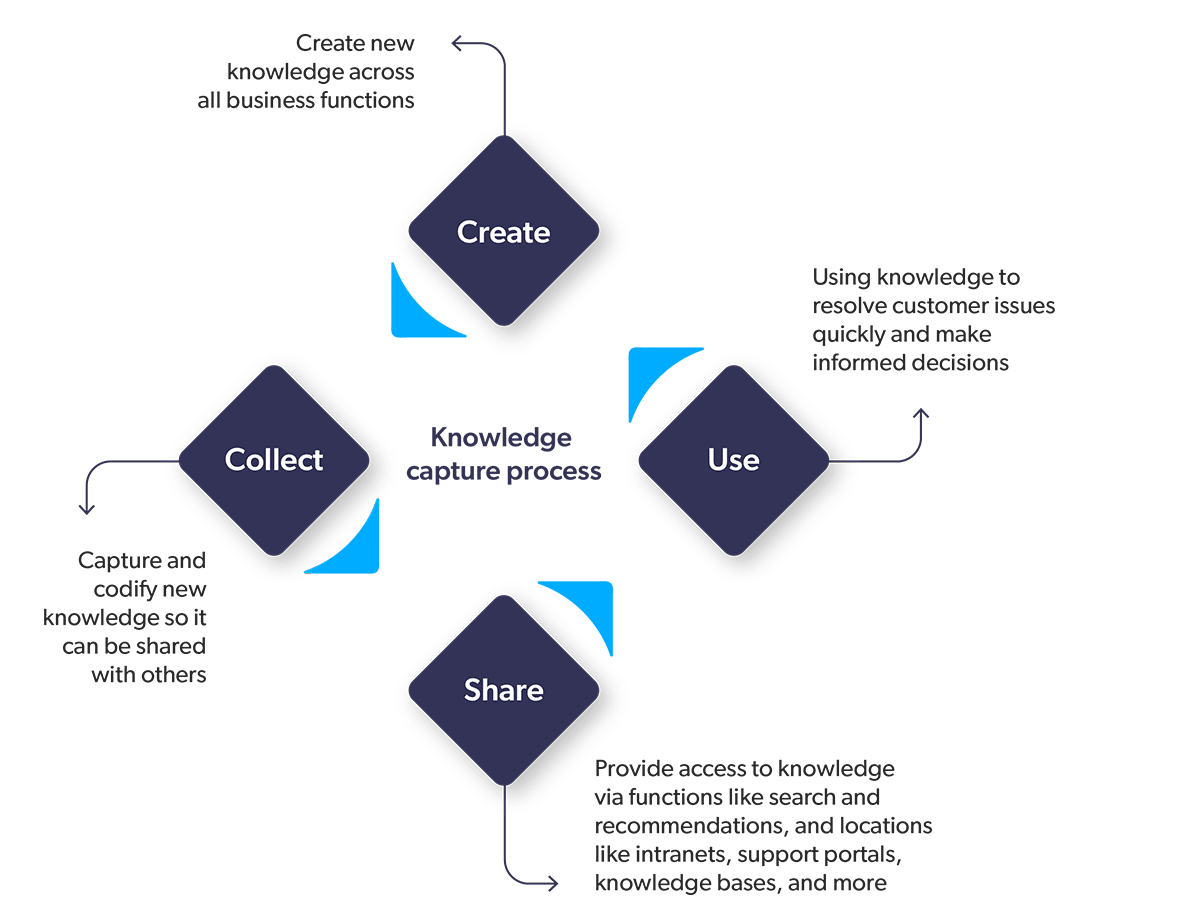
“This is a great time to lean into your knowledge program or start one,” said Neil Kostecki, Coveo’s VP of Product Service. “It’s the basis of your answers. Understanding language isn’t enough. You need to have the knowledge, culture, and the content to draw on.”
3. Keep an ear to the (digital) ground
Listening to customers is a key step in choosing an LLM that meets their needs. You should be asking things like:
- What tools are your customers willing to use?
- Do they want to talk with a chatbot?
- Do they want to interact with humans?
- Both?
You need an understanding of their tolerance for and acceptance of new technology or a new way of engaging.
LLMs also give organizations a new way to listen to customers. They’re able to make sense of large amounts of text. “We have a way of listening to customers like we never did before,” said Sweeney. “When they’re chatting in the community where they’re submitting feedback, we now have the ability to derive meaning from that.”
4. Invest in foundational technology like search
Think of intelligent search technology as a wrapper that can make generative AI enterprise-ready. Search is a way to activate generative AI, but it’s also a way to make your company knowledge — your content — do more heavy lifting. That’s why investing in search is connected to content creation. You’re investing in developing a language model that you’re then training to understand your domain.
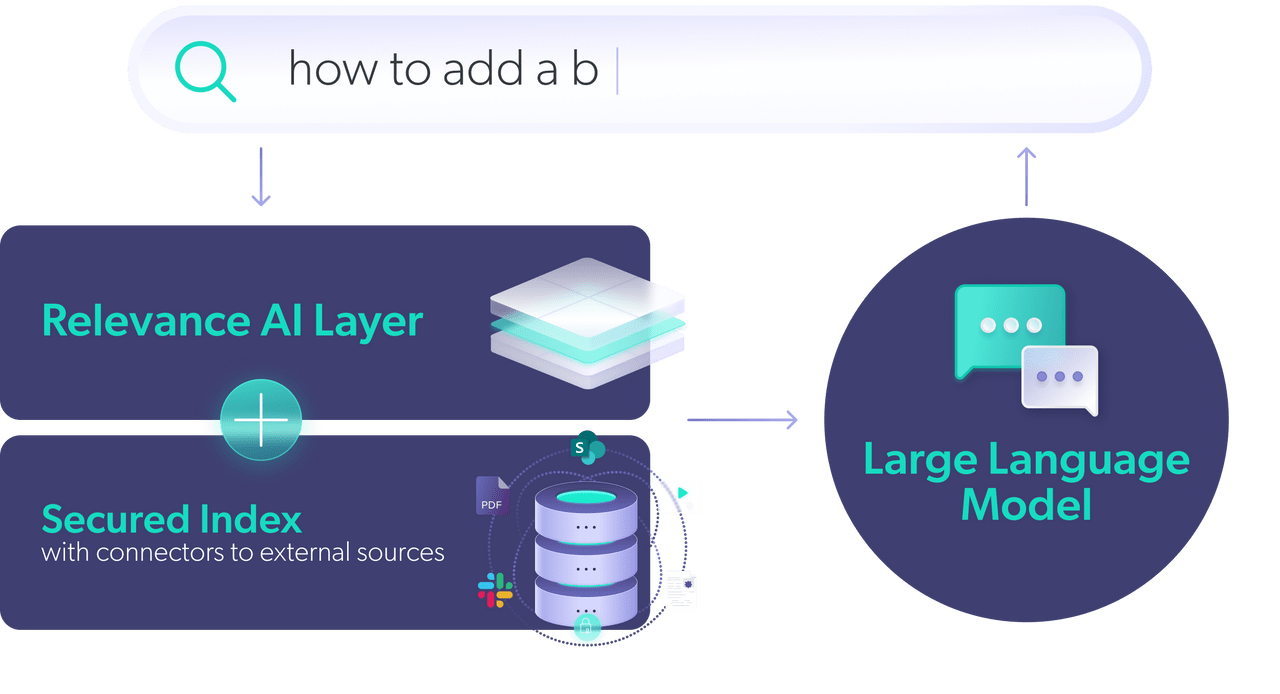
“Investing in search is not just about throwing down some money for technology,” said Sweeney. “It means that you’re committing to creating a new way of interacting with customers through enabling technologies and you’re putting more investment and effort into that.”
In addition to the above four points, it’s important to build your knowledge program around a digital self-service strategy when thinking about how and where you’re going to serve your customers. Self-help tools are widely deployed and used. In fact, 71% of support demand is initially serviced through self-help channels. But while many self-help transactions provide useful information, only 22% of cases are fully resolved and deflected from assisted channels.
Generative AI has the potential to resolve more cases, but only if the knowledge is readily available. “You need to have all your information in one place,” said Kostecki. “You need to be proactive. It needs to be contextual.”
What’s Next For Generative AI?
Wouldn’t it be nice if we could shift the entire cost structure of a support organization from lots of bodies delivering answers to lots of bodies, creating, curating, and developing large language models to do most of the heavy lifting?” asked Sweeney.
This question gets to the core of what’s next for generative AI. It’s not about replacing humans, it’s about augmenting roles and focusing on what makes generative AI work in the first place — a solid foundation of knowledge.
“First of all, it’s about having that content and getting it unified, secured, and integrated,” said Kostecki. “There are probably lots of organizations that are going to try and take their entire knowledge base and build their own model, but there are a lot of things to think about to make this enterprise-ready. You want to bring this into your support portal, your website, or your SaaS product. How do you go about scaling at a level that’s secure enough, scalable enough, and repeatable?”
There’s no silver bullet when it comes to generative AI and there are many things to consider when looking at the technology. This includes leveraging extensive pre-trained models (e.g., don’t reinvent the wheel), fine-tuning for your specific use case, and fine-tuning for your specific domain adaptation.
Once you have a structure in place to leverage generative AI, there’s no limit to what’s possible. “When you’re talking about something like ChatGPT and trying to figure out how to use it or implement it, think about the use cases that you want to implement,” said Bonnie Chase, Coveo’s Senior Director of Service Marketing. “Then you can really start planning how to meet that need.”
Watch the full presentation for the complete discussion:
Dig Deeper
All of the above said, generative AI — and many other flavors of AI — aren’t going away anytime soon. It’s all about doing the research needed to understand how this technology can positively impact your business, your employees, and your customers.
With these considerations in mind, how do you decide if generative AI has a place in your customer interactions and contact center? Download a free copy of our white paper, Preparing Your Business for Generative AI.