Generative AI has stolen all of the headlines lately. For good reason. It’s not every day that a technology comes along with this much transformative power. And it’s not surprising that customer service teams are fixated on the implications of artificial intelligence (AI), if not outright concerned.
Before you let your mind drift to doomsday Skynet scenarios, remember that we’ve been here before. After more than a hundred years with autopilot technology, you still find pilots in every cockpit. Most passengers probably want them there, just like they want real surgeons at their surgeries — despite the introduction of HERMES nearly 30 years ago.
As goes jumbo jets and intestinal anastomosis, so does customer support. The field will see significant augmentation thanks to AI — very significant — rather than wholesale customer service automation and replacement. This is likely for a number of reasons, which we unpack below in our responses to the five hottest AI takes we’re seeing circulate the world of customer support.
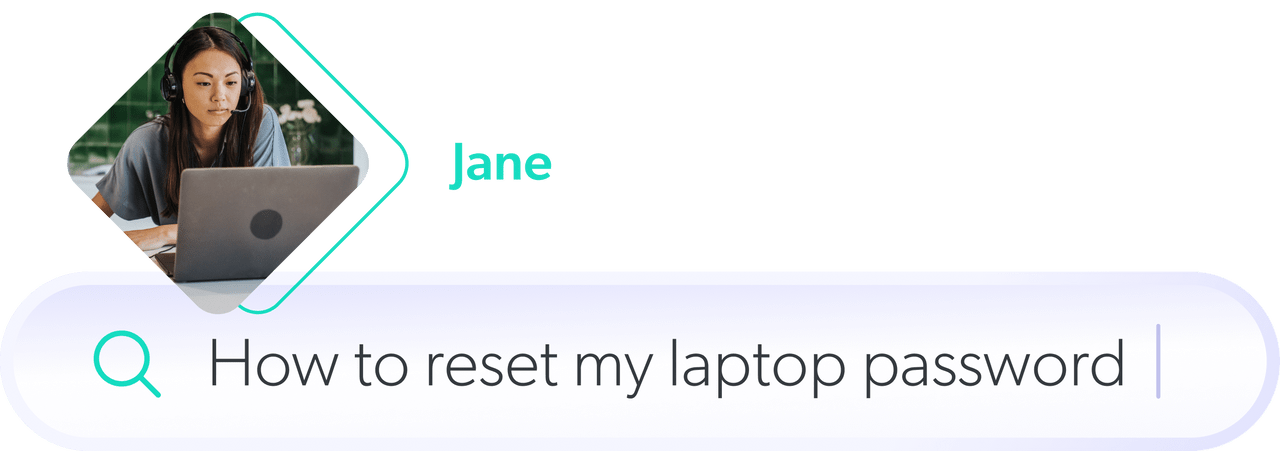
Large Language Model vs. Generative AI: What’s the Difference?
Large language models like GPT-3.5 and GPT-4 are trained on large data sets to analyze the structure of text. This enables natural language inputs and the ability to generate human-like responses.
On the other hand, generative AI involves the use of algorithms to generate new, original texts, images, or other media in response to prompts. ChatGPT, one of the more prominent examples, is a chatbot based off of GPT-3.5 and GPT-4 that simulates human-like conversations in a chat window.
Hot Take or Spot On? What Generative AI Customer Support Really Means
It’s easy to look around at all the concerned people and shrug it off. Relax, everybody. But generative AI really is unlike anything before and its power will have implications in the contact center. Very real ones concerning customer service automation, security, and jobs.
As to what’s true and what isn’t with respect to the future, well… It’s complicated, as these hot takes clearly indicate.
Hot Take #1: The Era of Customer Service Agents Is Over
While a lot has been made of the “death of support agents” at the hands of generative AI, remember that years ago websites represented the latest death knell for the customer service agent. Those websites ended up increasing contact volumes, as customers called in for password lockouts and help using the site.
Rather than the end of assisted support for customer inquiries, we’re likely to see the contact center teams who use generative AI replace the teams that don’t. The potential gains are too real to ignore. One Stanford and MIT study found that AI boosted agent productivity by 14% at a Fortune 500 company.
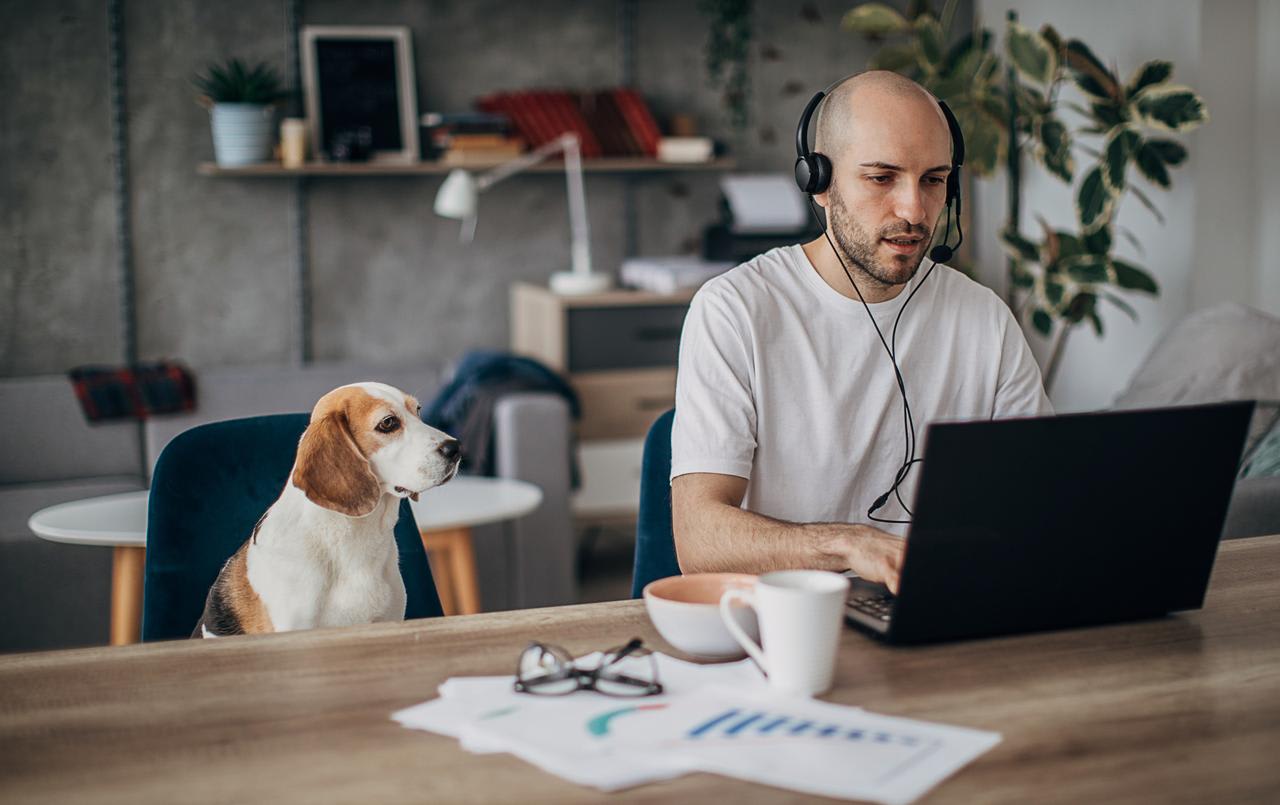
Much of the upfront support gains will come through an accelerated “shift left,” in which generative AI will handle more tier one issues on its own; anything new, nuanced, or more complex will be handled by agents. AI tools can give support agents insight to make informed decisions that are best for customer needs. This will also allow for an evolution in support and how customer engagement is driven.
Support Agent Knowledge Remains Critical to Relevant Support
These models are trained on a finite amount of data — they don’t know what they don’t know. In customer support, there will always be some new product, feature, and so on that requires new content (documentation, KB articles, etc.).
To make generative AI technology deployments as successful as possible:
- Prioritize content creation and capture: The large language model needs accurate and relevant content (especially areas of customer feedback like forums, small knowledge pieces, docs) to assemble cohesive answers; this can be provided via a unified index.
- Create new and adapted roles for knowledge management and agents: You’ll still need humans to edit and verify what the models piece together from different knowledge sources to ensure the best answers. Consider what your current knowledge management practices look like today, and how an AI tool can have an impact.
Hot Take #2: Generative AI Tools are the End of Search
As good as generative AI might be, it doesn’t solve for an age-old conundrum: most people don’t know what to search for or how. But they still need answers, and there’s a sweet science to giving someone a satisfactory answer they believe in.
When customers fully resolve their issues using self-service, three things tend to be true:
- They understand the information
- They trust the source of information
- They can confirm this is the best thing to solve their problem
In a word, customers feel confident. To build confidence in the answers customers get when using search supported by generative AI, start by showing your work. Customers want to see where content comes from — provide links to show what sources were used to generate the answer to their question. Use AI to guide the customer journey, too. For example, AI can help someone fill out a case submission form, or provide personalized recommendations based on what they’re typing into the search bar (in real time).
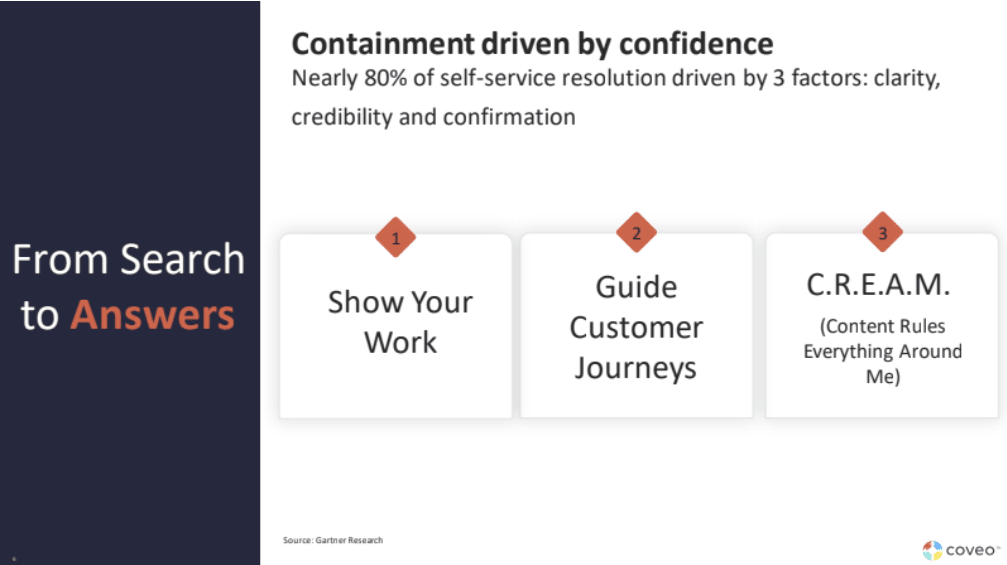
Hot Take #3: “We Can Build Our Own Generative AI”
The short answer to this hot take is yes you can. The question is, do you really want to? Think of generative AI investment like Tesla autopilot: it can be a great assistant, but you should keep your hands on the wheel for now.
Here’s three good reasons why:
Data privacy and regulatory compliance
Language learning and generative AI models are trained on collective information, and any information input can be surfaced to other users, leading to possible data leaks. Then there’s the difficulty in controlling what your teams put into the model, which Samsung found out the hard way.
Regardless, any model you (or anyone else) builds will be subject to various new legal requirements that are quickly evolving and that potentially restrict use cases.
Biases
Popular large language models are trained on the collective writing of humans across the world, past and present. This means that the same biases that exist in the real world can also appear in the model.
For example, ChatGPT has been shown to produce some terrible answers that discriminate against gender, race, and minority groups, something which its creator is trying to mitigate. Perhaps you’ve heard of the robots trained on AI that quickly became racist misogynists.
Training costs
While Gen AI is excellent at providing high-level answers due to its extensive training with numerous examples, it may not always be reliable in providing solutions for problems that are specific to your industry and business.
This training can be extensive and costly. You need to serve these models, maintain them, and provide fresh content — at scale, across your .com, chat, and other channels. It’s a big undertaking.
Hot Take #4: The Hallucinations Aren’t that Bad
Generative AI models don’t need lysergic acid to trip. They’re built on probabilistic language models that are prone to hallucinations of their own — that is, answers that sound correct, but can be self-referential and completely wrong.
Think back to when Google parent company Alphabet lost $100 billion in market value after the disastrous launch of Bard, which had trouble doing basic math. Then there are the many complaints of ChatGPT providing stats and data for which no known source exists.
Some people suggest that agents get it wrong sometimes, too, so we can live with these hallucinations. They’re not that bad. But when agents get it wrong, customer satisfaction (CSAT) plummets, escalations increase, and core metrics deteriorate.
What do you think will happen when your AI starts hallucinating? You don’t want it making things up inside of your knowledge base.
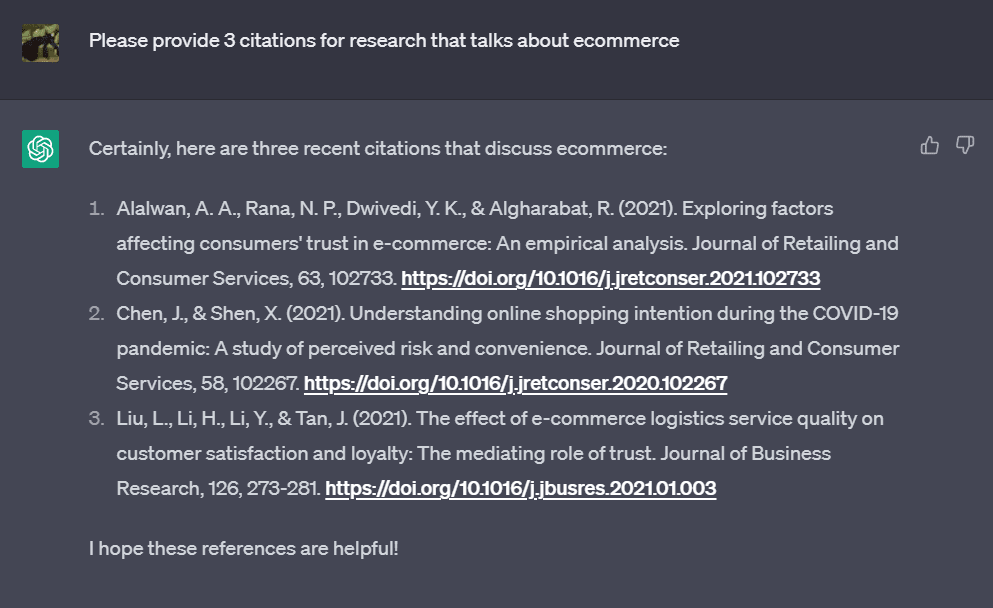
Hot Take #5: Internal Enablement is the Biggest Opportunity for Enterprise Generative AI Solutions
You can certainly augment the internal efficiency of your workforce by using tools that reduce repetitive and manual tasks. A few examples include Einstein-GPT, Slack GPT, and Github copilot. Many of these tools have shown the ability to reduce onboarding and time to proficiency.
Yet, people assume that the risks of generative AI are smaller for internal use cases, which isn’t exactly true. According to one cybersecurity firm, as much as 11% of what employees paste into ChatGPT is confidential. At the very least, companies exploring use of ChatGPT for customer experience should also evaluate use with employees.
Interested in Leveraging Generative AI in Customer Support?
All of the above said, generative AI — and many other flavors of AI — aren’t going away anytime soon. It’s all about doing the research needed to understand how this technology can positively impact your business, your employees, and your customers.
With these considerations in mind, how do you decide if generative AI has a place in your customer interactions and contact center? Download a free copy of our white paper, Preparing Your Business for Generative AI.