Generative AI is promising, and many people are testing it out. But it’s one thing for an individual to use ChatGPT to write folk songs about rust programming or using Google Gemini (formerly Google Bard) to create questionable images of America’s founding fathers. It’s quite another for businesses to integrate generative AI (GenAI) models into their workflows.
The big question many businesses are grappling with is, how can they make generative AI enterprise ready? Businesses like Xero are already using GenAI for customer self-service.
Others are enabling their employees to experiment with generative AI tools (in a secure environment), but most are still trying to figure out how to safely incorporate artificial intelligence and the right generative AI model into their business.
Relevant Reading: Generative AI & Top AI Models for Customer Experience
What Are the Key Features of Generative AI?
Generative AI is a form of advanced machine learning that is trained on large datasets and uses deep learning techniques to create new content like text, images, and video.
Key characteristics include:
- Requires training data: Generative AI models are trained on large datasets. They need big quantities of data to accurately identify patterns so that they can understand how to create new content.
- Recognizes patterns: In the context of AI, pattern recognition means the system can identify and understand relationships in the data “fed” to it. These patterns are what generative AI tools use as a foundation to generate new (original) content.
- Relies on neural networks: A generative model relies on a neural network – computer systems that mimic how the human brain processes information. This is kind of like thinking in that it allows generative tools to process data and identify patterns.
- Uses a large language model (LLM): Tools like ChatGPT and Bing Chat use LLMs for natural language generation.
- Gets faster: An advanced AI model like generative AI uses machine learning to improve their performance over time.
- Has many applications: Generative AI has near limitless application possibilities. It’s promising for marketing, sales, and product development, but also for customer service, IT, and programming applications. It shows promise in just about every business sector and category.
- Can be problematic: A generative AI tool is only as good as the data it’s trained on. It can produce inaccurate or completely fictional information. It’s been known to completely make stuff up – facts, sources, news headlines – a phenomenon known as “hallucinations.” It’s also prone to bias, again, based on the data it’s trained on. Finally, there’s a risk of plagiarism or copyright infringement in AI generated content, particularly if the model is trained on copyrighted material.
Enterprise Generative AI: Main Considerations
Generative AI’s unique ability to create human-like responses to natural language inquiries, create multiple iterations of images, and generate code (among other things), make it a potentially powerful tool for businesses.
But determining if your organization is ready for generative AI is hardly straightforward. To make these tools enterprise ready, you need to work with skilled data analysts, engineers, and machine learning experts to adopt and integrate generative AI in a way that’s secure and reliable.
Generative AI models also need a steady stream of feedback, a wealth of training data, and supervised learning to operate effectively. If you want to align generative AI with your specific knowledge base, product catalog, language, and brand voice, you’ll need to train a custom model. The financial investment to train these models — and maintain them — can add up to millions of dollars. (You also have the option to hire someone to do it for you.)
That said, GenAI has a lot of promise for business use cases like customer service and workplace scenarios:
- Help customer service agents and employees become more proficient; not by throwing a list of blue links at them, but by synthesizing multiple content chunks together into a coherent, actionable answer
- Surface knowledge for customers faster, so they have what they need to make well-informed decisions; which leads to a good experience, and cultivating long-term loyalty with your company
- Put knowledge managers on the front foot when it comes to identifying and mitigating content gaps; because users interact with GenAI using longer, more contextual prompts, this gives knowledge managers more insight into what their true intent is — and where their corpus might be lacking
Relevant Reading: 5 Hot Takes: How Generative AI Will Change Customer Support
5 Best Practices for Enterprise Use of Generative AI
It’s one thing to choose the best generative AI solution for your company, but quite another to implement it safely and effectively. When considering how to integrate generative AI work into your business, there are some best practices you should consider.
Strategically, it makes sense to create a generative AI strategy just like you would a marketing or sales strategy. Getting as specific as possible about how you plan to use Gen AI for things like image generation, conversational AI, and generative answering keeps you focused and allows you to be purposeful and minimize mistakes.
Best Practice #1: Choose a use case
Deciding on a business use case is a critical first step for implementing any new technology, but it’s particularly important for generative AI.
As we touched on above, there are many ways companies can use generative tools to enhance workflows. It’s easy to get overwhelmed or lose focus if you don’t outline clear objectives for the technology and create a plan – with measurable benchmarks – for using it.
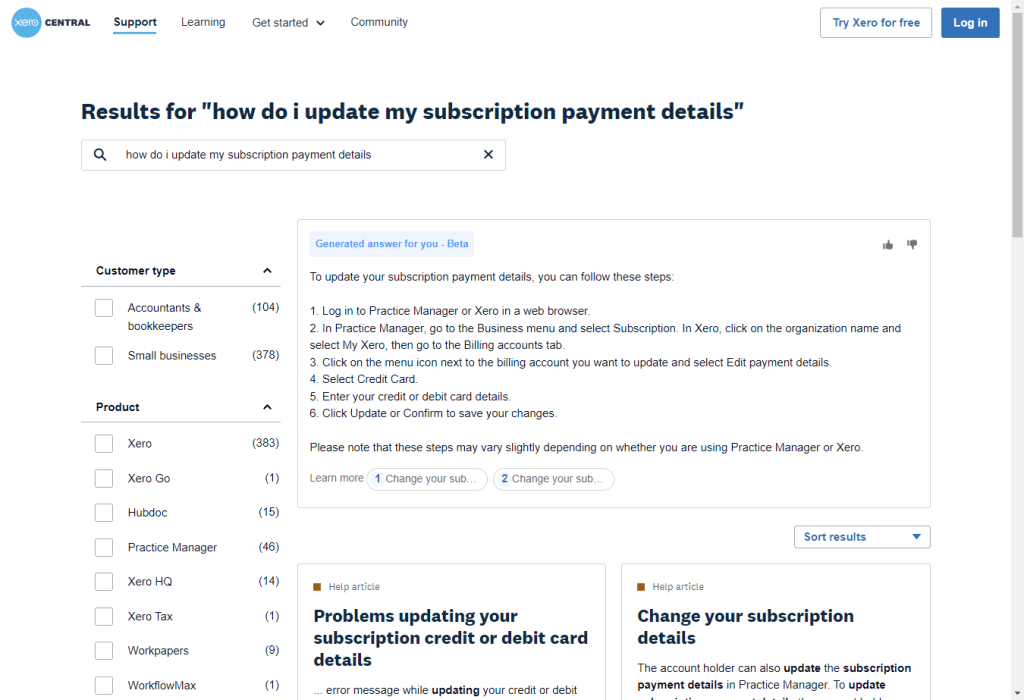
Modernizing enterprise search, one of Coveo’s core capabilities, is an example of how enterprises can use generative AI to effectively improve customer and employee experience. As an intelligent search platform, this is a use case we’re deeply familiar with. Using approaches like retrieval-augmented generation (RAG), generative AI-augmented search helps sales, marketing, and customer service teams locate and access information quickly.
RAG streamlines and safeguards generative answering by dynamically integrating information retrieval with natural language understanding and generation. Instead of merely returning links, RAG finds and supplies relevant documents or data to an LLM, which then synthesizes information for direct, concise answers, summaries, or insights. This not only improves the accuracy and relevance of search results but also offers a more conversational and intuitive digital experience.
Improving the efficiency and accuracy of generative answering across use cases is a key benefit of information retrieval techniques like RAG. This helps teams save time and quickly access knowledge when they need it. As leaders in enterprise search, enhancing search relevance and experience through the strategic application of generative AI is an area Coveo specializes in.
Relevant Reading: Your Blueprint to Generative Answering in Digital Self-Service
Best Practice #2: Establish data privacy guidelines
Generative AI algorithms need proper oversight to prevent data breaches, unauthorized access, and misuse of proprietary information. Creating a data governance framework to mitigate risk is an important best practice for companies that plan to use generative AI technology. This is particularly true as adoption increases with newer capabilities like generative answering. Having humans in the loop for training, testing, and case review will be critical for responsible AI deployment.
This is something that will likely require more expertise than you have in-house. Hiring legal and intellectual property (IP) experts can help you navigate potential risks and create a more comprehensive governance framework.
Some options are implementing human oversight for:
- Data labeling and annotation – ensures proper categories and labels are applied
- Results validation – humans check AI outputs catch errors and address ambiguities
- Exception handling – where AI encounters unfamiliar data, human expertise steps in
Following a rigorous human in the loop approach reinforces governance through greater transparency, higher accuracy, and ethical decision making.
Your organization’s leadership will also need to clarify and advocate for the responsible use of AI in your organization. To this end, creating guidelines that clearly outline what’s acceptable use — and what isn’t — helps to avoid issues of bias, plagiarism, and misinformation.
Best Practice #3: Test and learn
With all the hype, it’s hard to believe that generative AI is a technology that’s still evolving. Given this, we recommend testing it with different use cases with small, controlled test groups that allow you to evaluate its potential.
This not only gives your employees an opportunity to become familiar with different generative AI applications, it can help you identify specific use cases. Testing is also an effective way to understand the limitations of a given generative AI application. While it is a powerful innovation, it’s not perfect. It requires human oversight and careful monitoring to be effective.
Best Practice #4: Prepare your organization
Actively engage everyone in your organization in the process of integrating generative AI into your business operations.
You can do this by:
- Clearly communicating the importance of using AI responsibly
- Training employees on proper procedures, like
- Keeping data current
- Participating in continuous testing and feedback
You should also provide training on relevant topics like ethical AI practices, team-specific use cases, and the different AI technologies available and the terminology involved (e.g., What is a foundation model? How is data science related to artificial intelligence? What are generative adversarial networks (GANs) and do all generative tools use them?) Don’t expect people to understand how to use these tools – or use them well – without guidance and support.
An environment where team members feel comfortable reporting concerns, asking questions, and providing feedback is the best way to adopt and adapt a new technology.
Best Practice #5: Create a Center of Excellence
Technologies like Generative AI and predictive AI are transformative. At least, they have the potential to shake up the way we do business, but only if you (and your employees) are open to change. If you truly want to leverage and deploy these tools, you need to hire the right people – you may even want to invest in creating a center of excellence focused on discovering, integrating, and correctly using AI in your organization.
That doesn’t let other teams off the hook. Everyone in your organization should understand the implications for AI and be fully trained on how to use generative and other AI tools in their workflows.
But a skilled team that oversees AI initiatives can help you support all employees and departments as you embrace this innovation.
Relevant Reading: Reimagine Search With Generative Answering in Your Enterprise Tech Strategy
Leverage the Power of Generative AI Today
Coveo works with clients to integrate enterprise-ready generative AI into their organizations. Our AI-driven search and recommendation engine enables you to deliver highly personalized content and product recommendations to customers.
Built on your own data and run using enterprise-grade security, Coveo’s generative answering engine uses mature LLMs to identify relevant information in your documents and tailor its response to each user. Interested in learning more? Reach out to discuss how Coveo’s generative AI solutions can work for your enterprise.
Dig Deeper
How do you decide if generative AI has a place in your customer interactions and contact center? Download a free copy of our white paper, Preparing Your Business for Generative AI.
Relevant Reading: 3 Key Steps to Getting Started With Generative Answering