In a recent bombshell announcement, Google revealed that it has abandoned its plans to deprecate third-party cookies, finally scrapping its tumultuous, years-long effort to eliminate third-party cookies on Google Chrome. The upshot is that Chrome won’t join the ranks of Safari and Firefox in blocking the web trackers by default. Instead, it will introduce a new experience that lets users make “an informed choice that applies across their web browsing.”
In this post, we give a refresher on third-party data, why it matters, and explore how privacy-aware personalization can be effectively implemented, ensuring that your Search and Product Discovery strategies align with these evolving standards.
Privacy-First
Although Google’s update was light on details about what comes next, including a timeline for implementing new controls, it raises an important question: What does this mean for Search and Product Discovery personalization strategies?
The bottom line is clear. A consented first-party data strategy remains the most important focus strategically and will continue to play a growing role in personalization.
The overwhelming sentiment in the industry is for privacy-first technology. A third-party cookie is typically just a technical mechanism for sharing data, and the global regulatory tide for data sharing is still clearly trending towards more protection in terms of transparency and explicit consent for end users.
In fact, as a reminder of the general sentiment and regulatory direction towards increased transparency, and the risks in terms of brand reputation and compliance, theU.S. Federal Trade Commission has recently launched a study of products that could allow companies to set different pricesfor consumers based on their locations, past purchases, and other personal data, to personalize pricing for customers.
A Refresher on Third-Part Cookies and Personalization
The third-party cookie has long been a major player in ecommerce personalization strategies. But with many browsers now blocking (or giving controls to block) this type of cookie, brands and retailers must begin to look elsewhere to develop effective user personalization with privacy in mind. Let’s explore the different types of approaches, as well as which support privacy-first strategies.
First-party cookies vs third-party cookies: What are the differences?
- Third-party cookie. Third parties leave small pieces of data called “cookies” on a user’s web browser after a site visit. Third-party tracking cookies collect user data enabling the tracking of user behavior across the web. These are used heavily by digital advertising companies to create targeted ads. For instance, a google ad will create a cookie on a user’s web browser that will then monitor user activity on multiple websites. This behavior data is used to show those users product suggestions or further ads for products they searched for on different websites.
- First-party cookie. These cookies are website-specific. A website will directly place a cookie on the user’s browser when they visit to collect first-party data about their behavior on that website. This cookie type, also called “performance cookies” typically contains very detailed records and analytics for each website visitor. That data is then used by the website owner to improve their user experience. For instance, Google Analytics uses these cookies to capture data about web visitors and various aspects of the user experience.
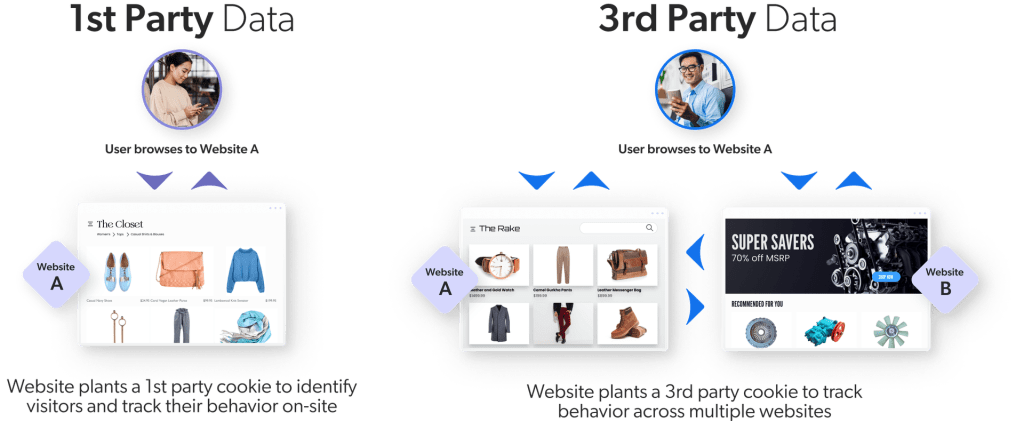
The Personalization vs. Privacy Paradox
Historically, data acquired through third-party cookies was a critical piece of any personalization strategy. This wealth of data allowed ecommerce companies to fine-tune each user’s customer experience based on their activity across the web. It enabled, among other things, digital marketing targeted advertising by leveraging user’s personal data.
However, Google’s recent announcement won’t stop the privacy revolution, driven by a global trend towards explicit consent and transparency. This shift reinforces the need for robust, privacy-first personalization strategies.
Now, this introduces tension. Shoppers want their experiences to be highly personalized, but they don’t want their data to be misused to serve corporate business interests. Cue thepersonalization vs privacy paradox.
Coveo’s own research shows that consumers have mixed feelings about sharing data for a more personalized experience when shopping online. While 71% of shoppers expressed concern over the use of their personal data, 65% said they were happy to do so with a brand that they trust, or if it means they get better deals and offers.
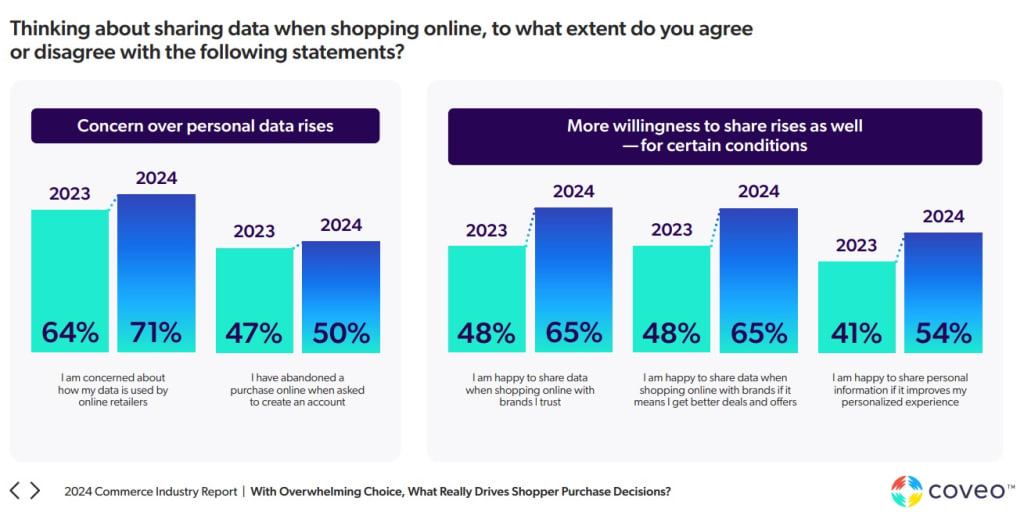
Will the Real Privacy-First Personalization Please Stand Up?
Brands and retailers don’t need to despair. Just because personalization initiatives can’t use browsing data from multiple sites doesn’t mean that companies must resort to guesswork. There is an alternative.
Companies that wish to embrace privacy-first personalization without compromising on relevance should focus on a first-party data strategy.
How Do You Leverage First-Party Data to Capture Shopper Intent?
First-party data is best characterized as behavior-based data associated with a tracking cookie. This data contains the course of a user’s movement on a company’s website providing very detailed records of what visitors do when navigating an e-store. With first party data, brands and retailers can glean valuable indicators into an individual’s interests and intent. Every user click is a potential opportunity for contextual advertising which provides the user with specially selected information.
First-party data can support contextual personalization which is a good starting point when tailoring experiences for your customers. This kind of personalization is based on referral data, local weather, and sometimes geo-location. However, it won’t capture the customer’s current intent, only the context in which they’re browsing on your website.
Shopper intent can be inferred from your shoppers’ onsite behavior, which is captured by first-party cookies. Retailers can study how users move around the site, what they click on, add to their cart, and how long they hover the mouse near objects. These clues can effectively work as windows into a shopper’s mind.
Leveraging visitor signals within a browsing session is particularly useful when users visit a site for the first time, or when they simply choose to remain anonymous. This is known as a ‘cold-start’ scenario.
Make no mistake — cold start problems are more frequent than one might expect.
What is the Cold Start Problem?
The term “cold start problem” actually originates from the automobile industry — when it’s really cold outside, a car engine has difficulty starting up, but once it warms up, it runs smoothly. Similarly, the best recommendation systems excel at guiding users to well-received options, but they often have difficulty serving new users. Let’s review a few types of cold-start problems.
In the world of ecommerce, a classic version of the cold-start problem refers to the difficulty of recommending products to genuinely new users. This is a significant challenge for brands and retailers, as a very large portion of the transactions taking place on their websites involve new users.
A cold-start scenario can also refer to when user data is available yet no longer relevant. Consider how data about a backpacking trip you took last year may no longer be relevant to the upcoming family-friendly trip with your children you’re shopping for. Or how a student looking for a bed may buy a twin mattress when sharing space with a roommate — but a few months later upon graduating, may opt for a queen instead to furnish a new apartment.
Finally, cold start problems can also occur when users have different interests at different yet closely spaced points in time. For instance, depending on their mood or their social context, users may be interested in watching different movies.
These are all kinds of cold-start situations where focusing on real-time intent and leveraging in-session clickstream data can prove extremely helpful.
First-Party Data Personalization at Work
In all of the above cases, personalization based on first-party behavioral data can prove extremely effective in deriving shoppers’ intent from user behavior. Because this might still sound abstract, we’ll consider a couple of examples to show how privacy-first personalization can actually work using first-party data.
Session-based Recommenders
The first example concerns the rise of so-called session-aware recommenders that leverage AI. These provide personalized recommendations by understanding visitor intent based on interactions observed in an ongoing session and analyzing the search and browsing behavior in real-time. For example, imagine I’ve searched for some golf pants on an ecommerce website, which I’ve promptly added to my cart.
Session-aware recommenders may start providing me with golf-related items automatically. This is a key feature and a game-changer that helps ecommerce players deliver personalized shopping experiences without the need for high volumes of data or logged-in users. These session-aware recommendations must remain relevant throughout the visitor’s session by also detecting intent changes and adjusting accordingly. For instance, you wouldn’t want to keep seeing golf accessories once you start shopping for your next tennis outfit.
To this end, Coveo has recently developed cutting-edge technology to provide Personalization as-you- go, using shoppers’ signals to tailor and improve the experiences that customers receive in real-time.
What to do When First-Party Data is Unavailable
But what do you do when site visitors don’t click “accept” the collection first-party data altogether?
First of all, only a low percentage of people actually block first-party cookies. By rejecting them they are telling you they have no expectation of personalization. Second, when first-party data is not an option, then you have to make do with experiences that are not personalized in-session but that are nevertheless hyper-relevant. For example, if it is not possible to serve session-based recommendations, it is still possible to offer product and content recommendations leveraging any model that is based on global data (popular products, trending products, new products) or that is based on product-to-product relationships (complementary products or similar products). These can still function without cookies and personalization.
While not as effective as personalized recommendations, “cookieless recommendations” can be used to showcase your store’s most popular products. These recommendations can help new shoppers save time, giving them access to some of the most valuable and potentially relevant products.
But, even these solutions come in two flavors – with Machine Learning (ML) generated recommendations or without. Vendors that offer ML-powered recommendations will have far greater relevancy than those that are simple rule-based. So even when first-party cookies cannot be leveraged to deliver personalized experiences, it is critical that websites still deliver experiences that are easy, effective, and relevant.
In Summary
Brands and retailers that wish to embrace privacy-first personalization without compromising on relevance should look for a technology vendor that can create personalized shopping journeys with first-party data and sophisticated intent detection technology. Machine Learning that can quickly detect intent based on a visitor’s interactions and tailor experiences for “cold-start” shoppers is an especially helpful feature to consider.