Let’s say I offered you the chance to improve customer experiences, support employees and the future of work, and significantly reduce app development costs. Let’s also add enabling machine learning capabilities (without tapping into your data science teams), reducing legacy technical debt, and addressing knowledge gaps exposed because more employees work remotely.
Would you take my offer and ask an internal team to conduct a proof of concept around this technology capability?
You can accomplish all of these goals with enterprise search in digital transformation programs, and recent research of tech professionals points to why more IT and digital leaders should prioritize this platform capability. Specifically:
- Gaps in knowledge access for remote employees is a top internal driver, and more customers relying on digital channels is a critical external factor. These are two reasons why 85% of respondents from large and medium businesses have increased their investments in enterprise search technology over the past 12 months.
- Businesses can target improved experiences and cost savings by investing in enterprise search. A cost savings opportunity stems from the 62% that manage multiple indexes for different applications, and experience improvements are opportunities for the 56% who acknowledge significant requirements changes from current capabilities.
- Many organizations report implementation challenges such as optimizing results (56%) and managing the search infrastructure (54%).
- While 93% of respondents believe AI will drive the future of enterprise search, 59% report struggling to find AI talent, and only 15% have implemented AI in their search stack.
If you’re a CIO or IT leader, these should be compelling reasons to take me up on my offer and conduct a proof of concept on an intelligent enterprise search platform. In fact, Perficient, a Coveo partner, helped a customer successfully implement AI-enabled search capabilities that removed the need to manually maintain 14,000 rules in their prior platform.
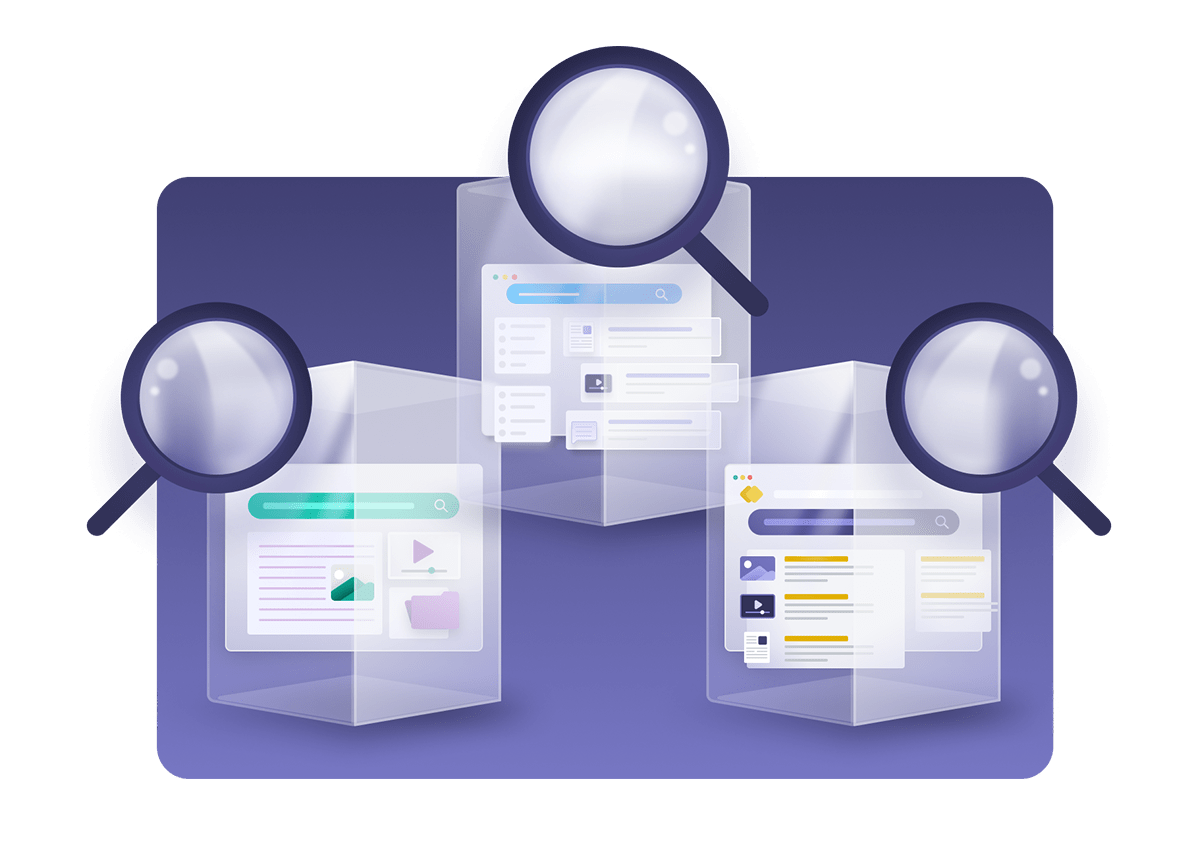
Legacy Search Tech: Platform Provides the Index, You Do All the Work
The problem with many available search technologies is that they aren’t platforms—they’re engines. These search technologies provide the APIs to create search indices, update them with new content, search them, and rank results. If they aren’t SaaS, then you have the infrastructure to maintain, and if accurate results are important to your business, then you probably have a dedicated development team to optimize relevancy and improve search experiences.
I recently shared my experiences with do-it-yourself search engines, which worked great for my business back in the day, spanning my CTO and CIO career from 1996 through 2016. On top of the indexes, we developed the code to enrich content, load content to the index, process search queries, and optimize result sets. Other advanced capabilities like recommendation engines, personalization, related content, faceted search, mobile experiences, and natural language querying required additional coding and engineering.
Development was just the start. In addition to ongoing enhancements, we had yearly major upgrades to the search platform, requiring extensive testing to ensure consistent or better results. Adding new content sources, deploying updates to the taxonomy, or revamping user experiences almost always required a major development effort.
Today, there are smarter, more effective, and significantly more efficient ways to implement enterprise search—especially if you’re like many larger businesses that have multiple application-specific and underperforming search technologies.
Reducing Tech Complexities in Developing and Enhancing Search Experiences
So, what’s different today that enables CIOs to build, deploy, and enhance world-class search experiences with less upfront and ongoing expense? Here are some of the capabilities without the tech, integrations, and data science complexities:
- Today’s search platforms support both employee and customer-facing search experiences. That wasn’t always the case, and many enterprises have multiple search technologies supporting these use cases.
- We tend to think of customer-facing search tied to ecommerce and content management capabilities, but there is a secondary use case to enable customer service with self-service capabilities, chatbots, and case management. Why shouldn’t customer service functions leverage the same knowledge stores and search capabilities rather than selecting a different technology?
- Leading enterprise search technologies offer out-of-the-box integrations with common CRM, CMS, community, customer support systems, ecommerce, productivity, cloud databases, and other platforms that store data required for customer and employee-facing search.
- Loading the content is half the battle—enriching it to enable relevant search results is another layer that used to require development and integration with content enrichment platforms. Today’s search engines offer real-time profile enrichment and use context-specific signals when personalizing results.
- If you thought it required too many experiments and iterations to optimize a user experience or get stakeholder consensus on a UI design, now think about all the back-and-forth needed to optimize search results. Automatic Relevance Tuning is a machine learning algorithm that analyzes user behavior patterns to optimize relevance ranking. Then add in other machine learning capabilities like dynamic faceting, recommendations, and query suggestions, and you’re getting an under-the-hood data scientist optimizing results with your content and data.
- Enterprises once had to choose and either select search appliances with limited customization options or assign a software development team and code on top of search engines, NoSQL databases, or columnar datastores. Today, IT can implement both paradigms with one platform that supports low-code and pro-code development options. Teams can prototype with low-code and then shift to pro-code implementations when the business case and requirements steer toward a more customized experience.
In summary, CIOs can get a platform to support multiple business functions, enable advanced machine learning capabilities, and leverage the appropriate development paradigm for the job.
Now, are you ready to prioritize that POC?