As the conversation around artificial intelligence (AI) evolves, one truth remains undeniable: clean data is the cornerstone of any successful AI implementation.
In a recent webinar titled “Clean Data, Smarter AI: The Impacts of Knowledge Management on Generative AI Effectiveness,” hosted by Coveo’s Senior Director of Product Marketing, Juanita Olguin, and Dan Shapiro, Associate Director of Enterprise Knowledge and Content Management at Organon, the critical importance of knowledge management was brought to the forefront of AI discussions.
It’s a topic that resonates with any business investing in AI, as companies discover the hard way that even the most sophisticated generative AI models falter without well-curated data.
Beyond the Hype: Where Generative AI Stands Today
Generative AI (GenAI) has gone through an intense hype cycle, with many organizations quickly adopting AI technologies, sometimes before they fully understand the complexities behind them.
As Olguin noted, “We’ve almost gone full circle on the GenAI hype curve.” Early adopters hoped for transformational results, but as the dust settles, many enterprises are finding themselves asking the same question: Does it actually work?
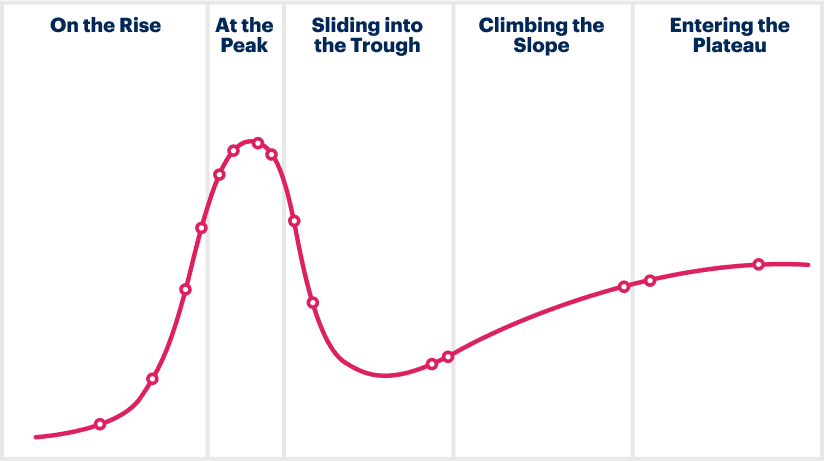
Olguin cited Gartner’s 2023 report, which predicted that more than 50% of enterprises building their own large language models (LLMs) would abandon those projects due to the high complexity and technical debt involved. This statistic underscores a harsh reality: building AI systems is hard.
Without a strong foundation of clean, well-structured data, businesses are more likely to hit roadblocks and fall short of realizing AI’s full potential.
The Complexity of AI: Why Knowledge Management Is Non-Negotiable
One of the key reasons businesses are struggling with AI adoption is the complexity of implementation. As Olguin put it, “There isn’t one silver bullet. Rather, it’s a series of components and elements that work hand in hand.” This complexity is why knowledge management is so crucial. AI needs structured, well-maintained data to function properly, and without it, the entire system breaks down. As the old saying goes, “Garbage in, garbage out.”
Shapiro, who has extensive experience managing enterprise search and AI at Organon, reinforced this point, stating, “You still have to have good foundation content. If not, if you have bad content, you’re just going to get bad AI out at the end of it.” His experiences underscore a common problem with AI: when data is outdated, irrelevant, or poorly organized, the AI struggles to produce meaningful insights.
He shared an example where Organon’s AI model pulled information from a folder titled Obsolete Files. This issue arose because the AI couldn’t differentiate between current, useful data and obsolete content that should never have been included in the first place. This example demonstrates how foundational KM is to AI’s success. Without clean, well-organized data, AI will surface irrelevant or outdated information, making it more of a hindrance than a help.
Relevant Reading: Are These KM Myths Weighing Your Company Down?
Why Search and AI Are Inextricably Linked
A critical takeaway from the webinar is the deep relationship between search and AI. AI, at its core, is a highly advanced search engine, designed to sift through vast amounts of data and deliver insights or answers. But to do this effectively, it needs to be fed relevant, up-to-date information. As Olguin explained, “Search relevancy, the ability to filter, rank, and factor out the bad stuff, really comes into play.”
This is where knowledge management proves essential. A robust KM system partnered with a strong AI search platform ensures that the content AI interacts with is accurate, relevant, and up-to-date. Without that, the AI will inevitably return subpar results.
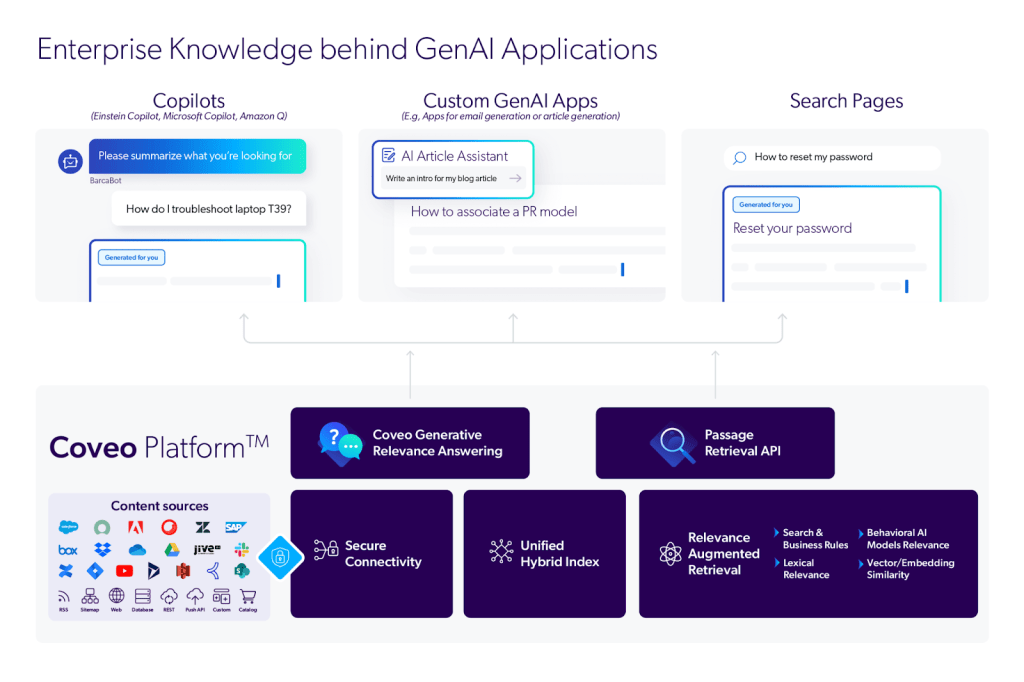
As Shapiro eloquently put it, “When we talk about AI nowadays, it’s really just a glorified search engine.” This isn’t to diminish AI’s capabilities but to highlight that its effectiveness is directly tied to the quality of data it’s searching through.
At Organon, Shapiro has worked extensively to ensure that their AI systems are supported by a solid search foundation. He described how the company leveraged Coveo’s AI-powered search tools to optimize results, noting that dynamic refiners and relevancy rules helped filter out irrelevant or stale content.
By investing in tools that ensure search relevancy, Organon has been able to maximize the effectiveness of its AI initiatives.
Relevant Reading: GenAI Is Only as Good as Your Search Relevance
The Need for Scalability and Flexibility in AI Deployment
Another major hurdle in AI adoption is scalability. While many companies can get AI up and running in small, controlled environments, scaling those solutions across the enterprise presents a significant challenge.
Shapiro shared how Organon navigated this issue by taking a methodical approach: “We spent about six months rolling out our solution, just trying to figure out how to get it right.”
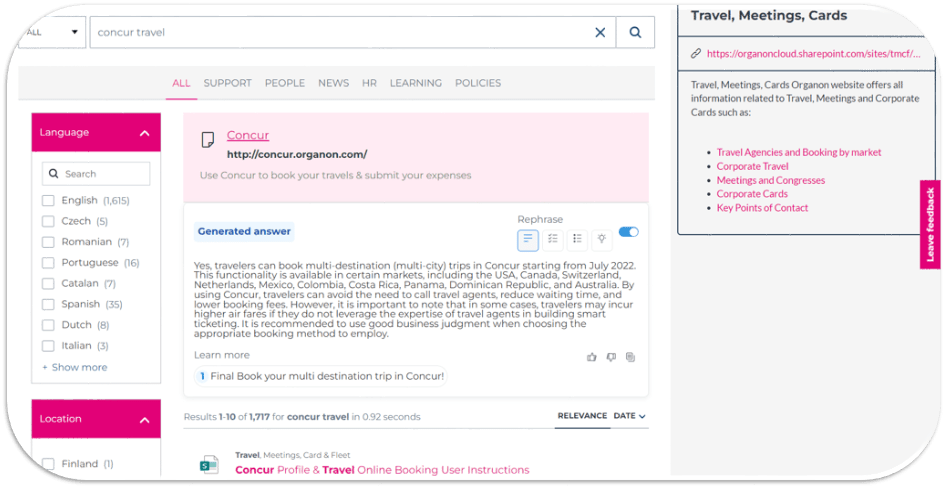
This careful approach allowed Organon to avoid many of the pitfalls other enterprises face when trying to scale AI. For instance, many companies rush through the proof of concept (POC) phase only to find that their AI models aren’t capable of scaling to handle the complexities of real-world applications.
The underlying issue here is often a lack of flexibility in the AI model itself. Shapiro noted that as new large language models are developed, enterprises need the ability to swap them out and integrate newer, more effective models into their systems. Without this agility, companies risk locking themselves into outdated technologies.
Relevant Reading: Your Blueprint to Generative Answering in Digital Self-Service
Clean Data Is Secure Data: AI and Compliance
In addition to scalability, security and compliance are paramount for enterprises deploying AI. Generative AI systems need access to a broad array of data to function effectively, but that data must be tightly controlled to ensure that only authorized users can access sensitive information.
Olguin stressed that Coveo takes security seriously, mentioning their certifications such as HIPAA compliance. This is an important consideration, particularly for industries like healthcare and finance, where strict regulatory standards must be met.
But security doesn’t just mean keeping unauthorized users out. It also involves ensuring that the data itself is clean and reliable.
As Shapiro explained, AI has the potential to uncover long-standing content issues that may have gone unnoticed for years. “GenAI is a funhouse mirror,” Shapiro said, “It will magnify the issues at a level you’ve never experienced before.”
In one instance, Organon’s AI surfaced content from old risk assessments that contained outdated policies. This led to quick action from Shapiro’s team to clean up the data and adjust the permissions, but it was a stark reminder that AI has the power to expose data management issues that might otherwise remain hidden.
Relevant Reading: Enterprise Safety: 5 Pillars of GenAI Security
Knowledge Management: The Unsung Hero of AI Success
All of these challenges — data quality, search relevancy, scalability, and security — point back to one core truth: knowledge management is essential for AI success. AI systems are only as good as the data they have access to, and without clean, organized data, AI will fail to deliver the transformative results businesses expect.
In fact, as Olguin pointed out during the webinar, companies that fail to invest in KM risk wasting their AI investments. “AI isn’t a magic bullet,” she reminded the audience, “It requires a strong foundation of clean data and knowledge management to work effectively.”
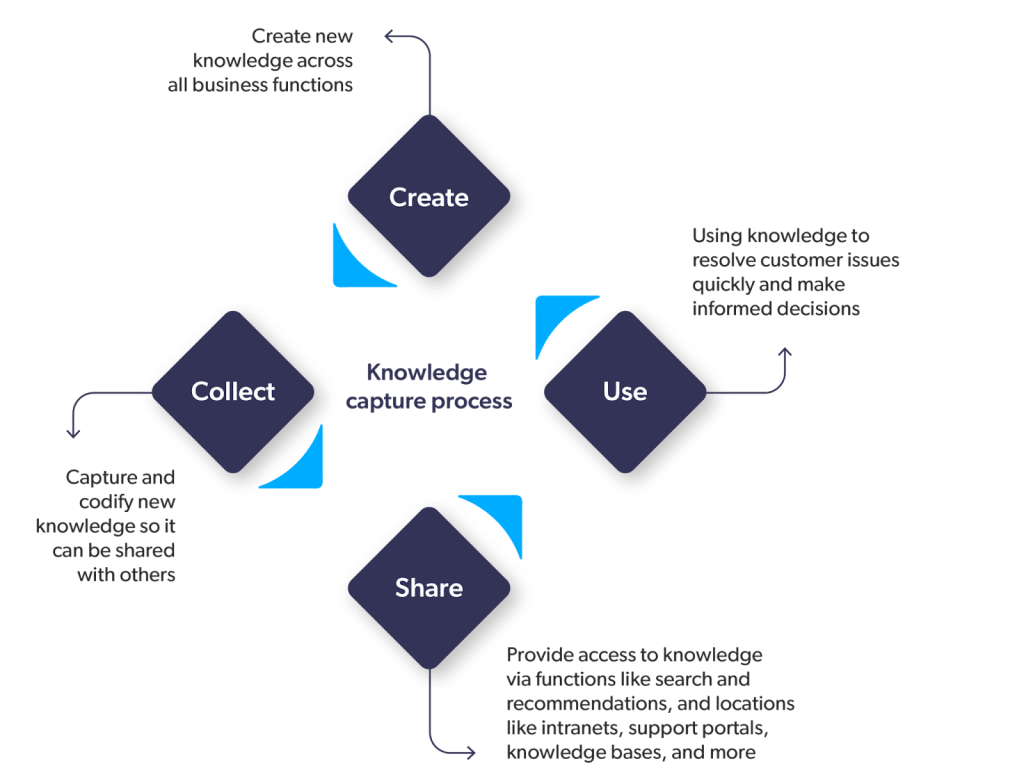
Shapiro echoed this sentiment, describing KM as the key to unlocking AI’s potential: “If you want to do search and AI well, you have to be doing the other stuff.
“You have to have KM processes. You have to have clean content.”
Relevant Reading: What’s Your Company’s Knowledge Management Maturity Level?
Realizing ROI: The Payoff of Clean Data and Effective AI
For companies willing to invest in knowledge management, the payoff is significant.
Shapiro shared that Organon has seen a marked improvement in productivity and employee efficiency since implementing Coveo’s AI-powered search and generative answering tools. By surfacing relevant information quickly and accurately, employees are able to spend less time searching for data and more time focusing on high-value work.
Olguin highlighted some key statistics to back this up, noting that customers using Coveo’s generative AI for self-service have seen a 20% improvement in case deflection. This equates to substantial cost savings for large enterprises that typically deal with high volumes of customer service inquiries.
These tangible results are crucial for getting executive buy-in, especially in industries where ROI is scrutinized closely. As Olguin pointed out, demonstrating concrete improvements in business outcomes is the key to justifying continued investment in AI technologies.
Conclusion: Investing in Clean Data for Long-Term AI Success
In the world of AI, clean data isn’t just a nice-to-have — it’s a necessity. As enterprises continue to adopt generative AI solutions, those that prioritize knowledge management and invest in maintaining clean, organized data will be the ones to see the greatest returns.
For businesses looking to implement AI effectively, the message from Coveo’s webinar is clear: there are no shortcuts. AI success is built on a foundation of clean data, robust KM practices, and a commitment to ongoing optimization. By embracing these principles, companies can unlock the full potential of AI and drive meaningful business outcomes in the process.
This blog summarizes the KMWorld webinar, Clean Data, Smarter AI: The Impact of Knowledge Management on GenAI Effectiveness. Watch the on-demand recording for all the insights:
Dig Deeper
GenAI is becoming central to CX strategies across platforms and industries, but it can’t do it all on its own.
Data cleaning can seem daunting, but our ebook highlights why it’s the overlooked driver of better search experiences and company success. We broke it down to the 6 best practices.
Offering your customers the most relevant results to boost engagement and retention begins with serving them better information – the data you feed your LLM. Check out the ebook and move towards providing better search experiences.