The hype around tools like ChatGPT, Google Bard, and Dall-E that leverage artificial intelligence and large language models (LLMs) to create content and improve customer self-service is compelling. It’s hard to resist the urge to integrate a generative AI application into your business, particularly as various tools and models continue to be featured on major media outlets like CNBC and CNN. The news tends to focus on what’s exciting or controversial about the purported capabilities of generative AI solutions, but it’s important to separate reality from hype.
The big innovations driving generative tools include deep learning architecture like transformers, generative adversarial networks (GANs), and diffusion models for image generation. Different models are focused on specialized tasks like creating new content or recognizing images. Generative artificial intelligence is trained on massive datasets – entire libraries of information – that allows them to perform tasks that were once relegated only to humans. How these systems are built makes a huge difference in how they work.
Factors like what specific data was used to train a generative model, how the system gets tuned to perform different tasks, whether it uses synthetic data or not, and what it focuses on have massive impacts on the reliability and usefulness across different situations.
Before investing in generative AI for customer self-service, organizations should ask vendors key questions, including:
- What specific training data and tuning was used for the LLM offered?
- How does the machine learning model build in safeguards against bias, inaccuracies, or hallucinations?
- For a given use case, what proof exists that this LLM-enabled tool will be effective?
Thoughtful preparation is also key to successfully leverage generative AI:
- Identify high-impact business use cases where improved customer experience is the goal.
- Build a Knowledge Centered Service® (KCS®) content foundation to train solutions.
- Invest in foundational technology like intelligent search to integrate new capabilities.
Asking tough questions and laying groundwork will enable you understand if Gen AI is truly a good fit for your needs. Responsible AI solutions hold promise, but thoughtfully matching them to business needs is essential.
Improving Self-Service Success by Building Customer Confidence
Nearly 80% of self-service resolution is driven by three factors: clarity, credibility, and confirmation. With a generative AI model, it’s not just about the generated information being correct. You also want your customers to trust that it’s correct.
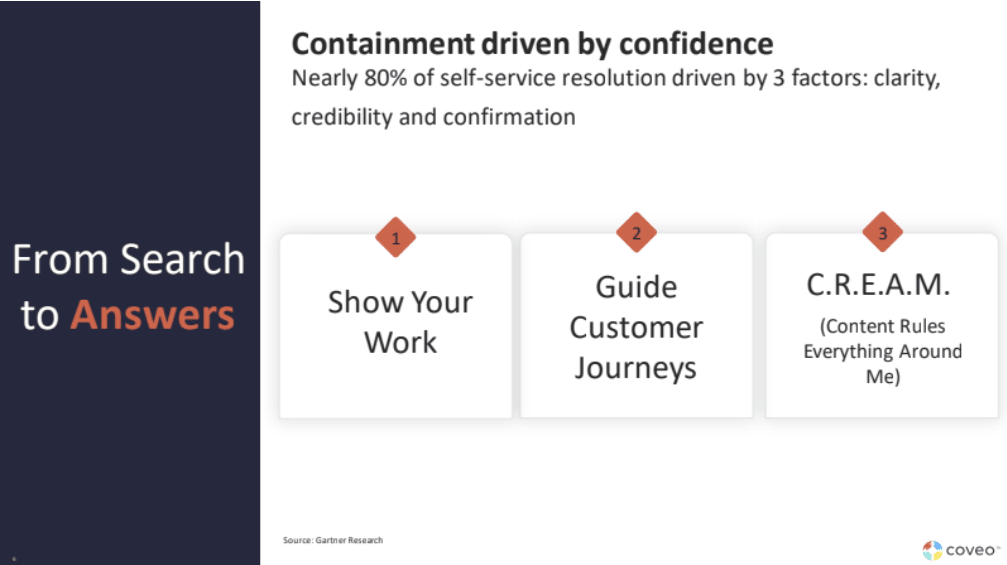
When getting customers comfortable with this technology, you need to consider the following three tenets:
1. Show your work
It’s easy to ask a tool like ChatGPT a question and get an answer that looks extremely accurate. But looks can be deceiving. A generative AI system, using deep learning and LLMs to craft a natural language response, can sometimes provide false information. When AI-generated content that’s false or pulled from bad sources is presented as factual or true, it’s called a “hallucination.” To avoid this, you need reliable input data, reinforcement learning, and an accurate language model. These are used to train any system you plan to use in an enterprise setting.
Allowing customers to check where an answer came from and the logic behind it can increase their confidence. Do this by providing the attribution and source links wherever possible. Then they can visit the source if they want to understand how an answer was assembled.
2. Guide customer journeys
Giving customers guidance on how to use generative AI technology can help improve confidence in their responses. It can also yield more relevant answers. There’s a learning curve with generative AI tools, which is why prompts can help users have more productive interactions.
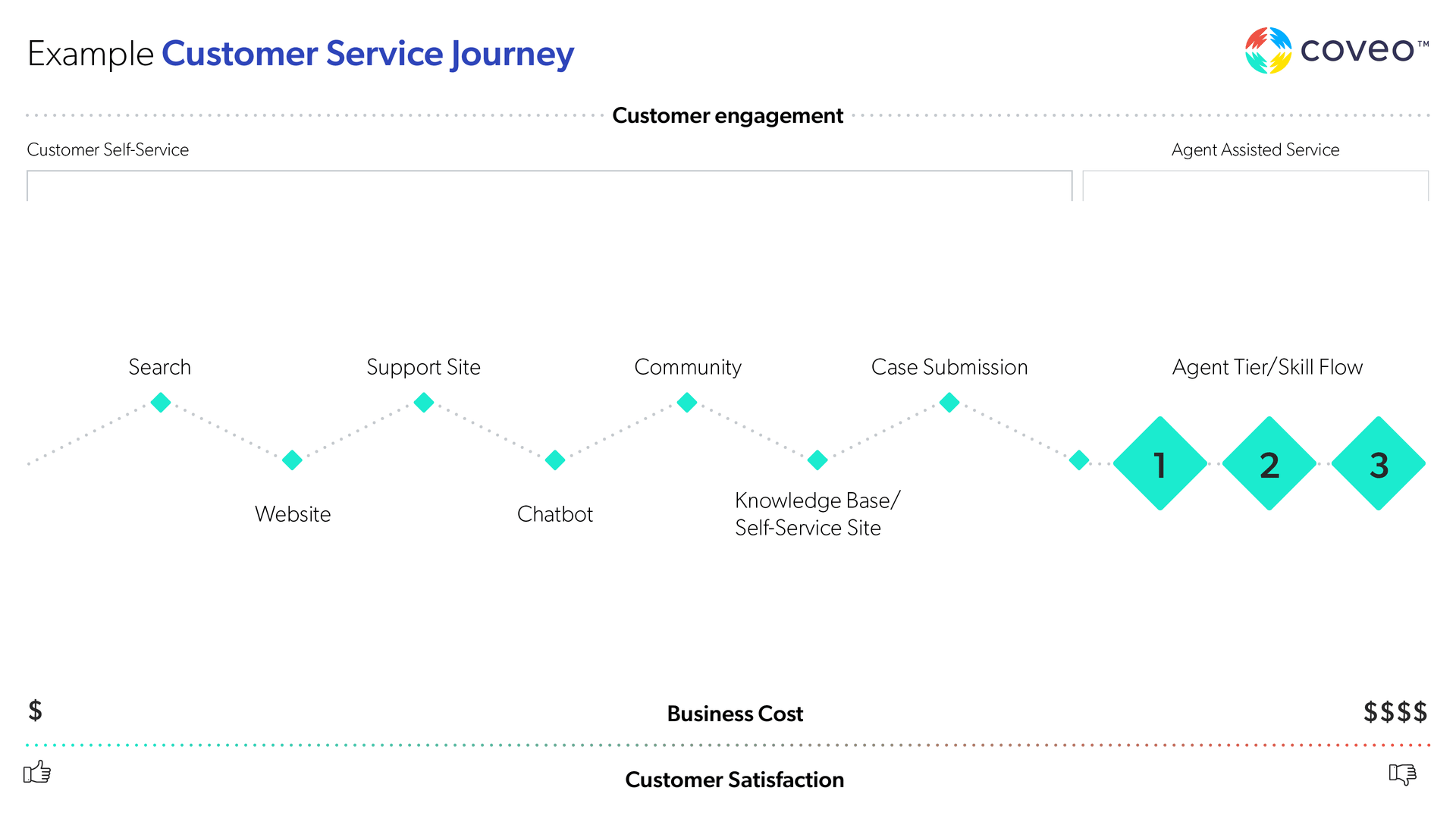
Generative AI and the technology behind what makes it work is something entirely new for many people. Guidance like visual cues, prefilled forms, or written instructions helps users understand how to interact with the technology. And, in return, this helps the generative AI model refine future answers.
3. C.R.E.A.M. (Content Rules Everything Around Me)
The quality of generated content is a critical part of what makes a generative AI solution successful. It’s not enough to have an answer that looks accurate. It needs to pull from high-quality sources to ensure that it’s factually and contextually correct.
Your company’s knowledge management process — and all the content that forms its foundation — is the baseline for generating answers. Without a solid understanding of your existing content, including a comprehensive grasp of product data and user interactions, your generative model won’t have what it needs to create reliable information.This is what we mean when we say “content rules everything around me.”
Coveo’s product embedding and vectors model is an example of how your content can and should inform a GenAI model. It maps out how products relate to one another using product embeddings. Simply put, machine learning is used to position similar products close together in a kind of “relationship graph.” Different products end up further apart. Mapping these connections allows the AI to better understand subtleties between products that let it deliver highly personalized, accurate recommendations and search results.
Essentially, the AI gets a cheat sheet detailing product relationships instead of trying to figure it out from scratch. Constructing this degree of understanding about how all the data interconnects takes some sophisticated intelligence on the AI’s part. It shows the level of comprehension required for reliable, generative AI.
This is one example of how your existing knowledge base is so critical. If you put an LLM on top of an insufficient knowledge base, it won’t yield helpful results.
8 Questions to Choose Best Generative AI Solution
When choosing a generative AI tool for your business, there are a lot of questions to ask. Some of these questions focus on the technology itself. Others help you boil down the best way to use these tools within your existing customer service framework.
1. Can generative AI eliminate the need for support agents?
No. Generative AI models are about augmenting, not replacing, human agents. For a frictionless customer experience, have a plan to manage the digital self-service to human interaction transition — and vice versa.
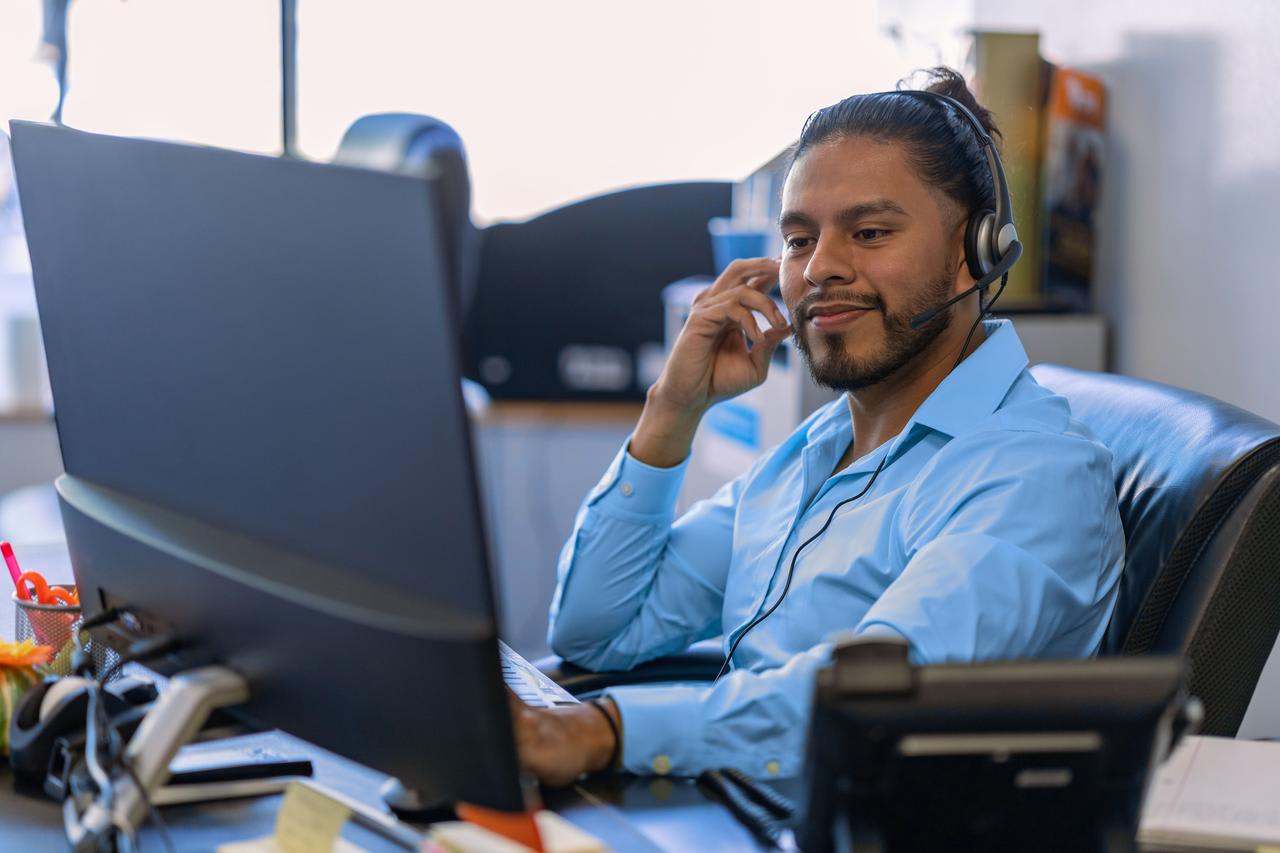
This could include:
- Instructions on when a customer should escalate an issue
- What AI tools like conversational AI chatbots can/should handle
- When an issue is complex enough to need problem-solving by an agent
No language model, no matter how good it is, will be up-to-date enough to know all the answers. It should be part of your AI strategy to understand how this technology fits into your existing service model. Ask about this and how generative AI applications can help your live agents-versus if or how it can replace them.
Relevant Reading: 6 Strategies for Improving Agent Experiences in Salesforce
2. How does a given generative AI tool support KCS?
Generative AI can provide more personalized, relevant answers in a fraction of the time of a traditional search. When reviewing vendors, ask how they can support your customers and employees to find the right information more quickly.
By synthesizing chunks of information across multiple documents, generative AI lessens the workload for those seeking an answer. Users — both customers and employees — can ask a natural language question and get a response that’s best suited for them.
If you work in an industry where information must be surfaced exactly as-is, generative AI may not be for you. Instead, Coveo offers another LLM-backed feature called Smart Snippets. It displays a relevant snippet of information on the search results page. Users can get an instant response to their search query or dig deeper by clicking on the snippet source.
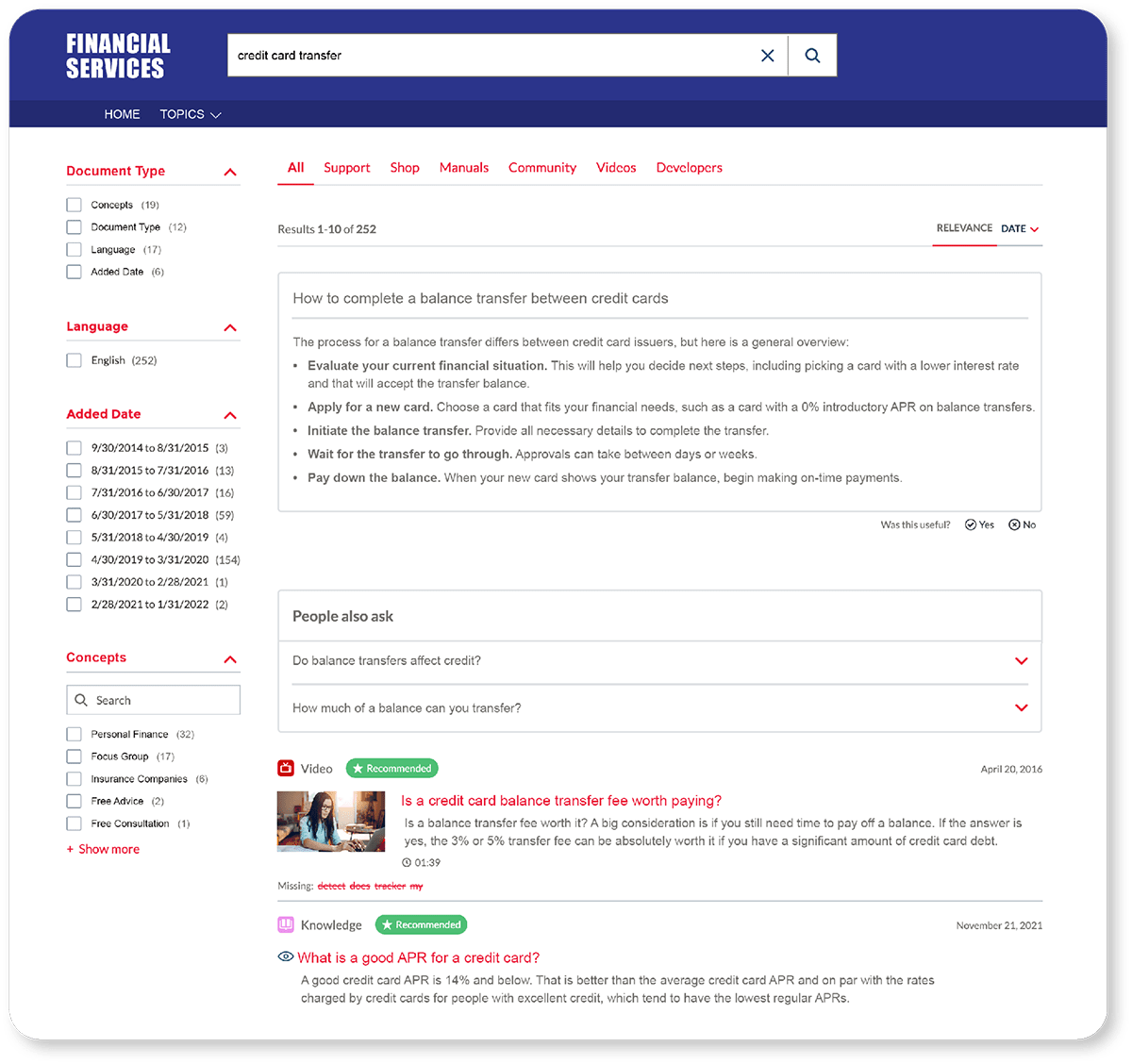
Relevant Reading: 5 Best Practices for Multichannel Knowledge Management
3. What is the timeline for implementation?
Integrating generative AI models like Coveo’s Relevance Generative Answering (RGA) into an existing service approach varies depending on multiple factors. From pre-implementation tasks like integrating the system into your existing workflow (e.g., portability) and tech stack to post-implementation fine tuning, like customizing a foundation model for a specific task.
The most successful implementations start with understanding the scope of the project and asking the right questions. For example, how do you integrate search, conversational AI, and question-answering together? How could a model like RGA contribute to the user journey? These questions can help you narrow choices for a solution that works for your use case and organizational goals.
4. Is this tool enterprise-ready?
Tools like ChatGPT that use LLMs and generative AI need maturity before they can be enterprise ready. Find out if there’s an administrative wrapper like intelligent search. As well as other tools that allow you to train and define an LLM to serve as a conversational agent. Additionally, explore if synthetic data can be utilized to enhance the data science aspect of training, ensuring a more robust and versatile application.
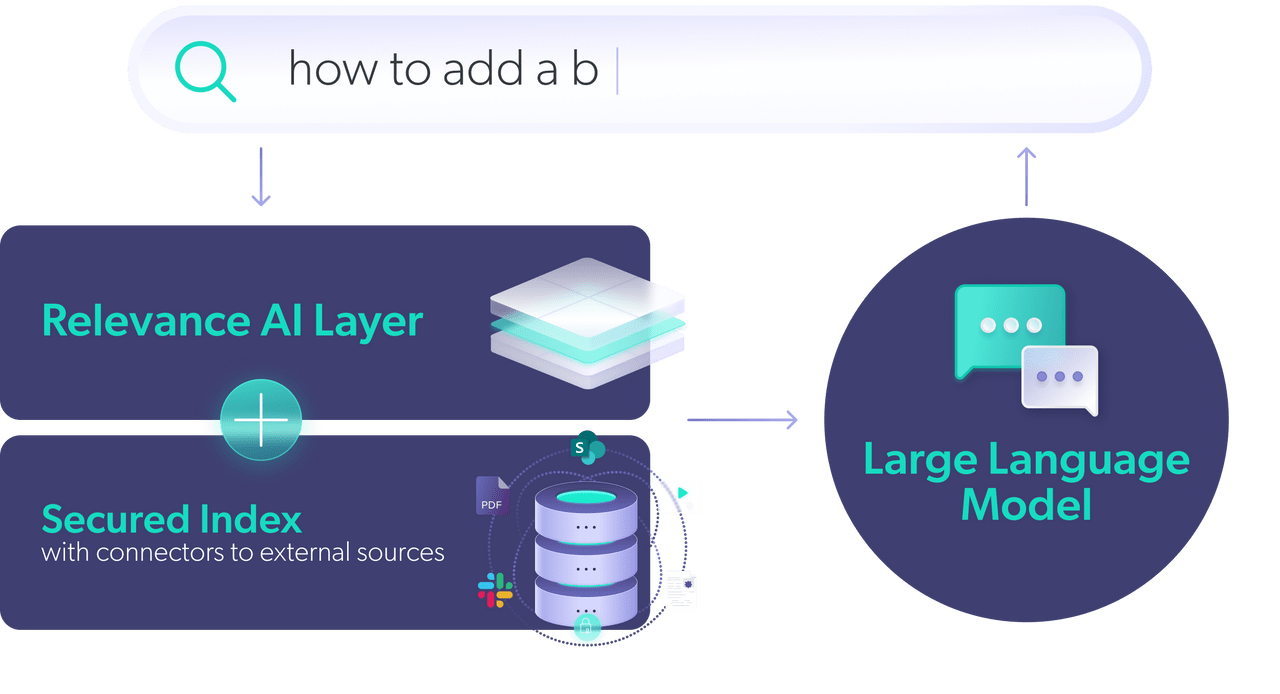
Investigate the scalability and maintenance of the solution. You’ll want to make sure that it can handle day-to-day requests without being interrupted. Ask about security measures and how they align to your IT policies. Not many IT departments will let you download code, throw it on the corporate site, and say “go at it.”
Relevant Reading: GenAI Headaches: The Cure for CIOs
5. Can the system leverage pre-trained models and can they be fine-tuned?
Many generative AI vendors provide pre-trained models to help you get up and running quickly. Pre-trained models can be used in the same way as self-training or custom training. However they are usually more limited in terms of customization and may not always fit into an existing service model. If a tool uses a pre-trained model, ask if you can fine-tune them for existing use cases and domain adaptation (i.e., using your vocabulary), potentially involving supervised learning techniques to enhance performance.
6. What is the required infrastructure?
You need the proper infrastructure to support ingesting and feeding the right context into an LMM. Open source models like sT5 and MPNet, which may incorporate techniques like diffusion models and generative adversarial networks, outperform mainstream GPT models in most real-world retrieval scenarios. It’s important to ask about the performance and operational efficiency of the LLM you choose within your generative AI system. Consider the appropriate neural network architecture given the cost/quality constraints to productize for hundreds of enterprise clients.
7. Can you build a test model?
Ask the AI vendor if you can build a test model. This should allow you to evaluate the performance of their technology with your existing data before you commit to it. You’ll get a realistic sense of how long it will take to train a model, as well as generated content accuracy and answer speed.
8. Is the retraining schedule appropriate and easy to change?
Machine learning models need to be retrained to avoid performance degradation. A retraining schedule is typically based on intervals of time (e.g., weekly, monthly, quarterly, etc.) They can be performance based, with retraining occurring when performance falls below a predetermined threshold.
Ask how the generative AI vendor approaches retraining — is it done manually or implemented automatically based on preset conditions? It should also be easy to change since the conditions and/or requirements of the tool may need to be updated to align with your internal processes and needs.
Choose the Right Generative AI Solution For Your Business
Generative AI is a new and exciting tool that will change how we consume information. Make sure you choose the right platform for your business by considering all your needs, options, and more.
Curious to see how Coveo is handling generative AI? Find out more here:
Dig Deeper
How do you decide if generative AI has a place in your customer interactions and contact center? Download a free copy of our white paper, Preparing Your Business for Generative AI.
Relevant Reading: 3 Key Steps to Getting Started With Generative Answering